Tactile Sensing is a crucial modality for intelligent systems to perceive and interact with the physical world. The Gelsight Sensor and its variants have emerged as influential tactile technologies, providing detailed information on contact surfaces by turning tactile data into visual images. However, vision-based tactile sensing lacks transferability between sensors due to design and manufacturing variations, resulting in significant differences in tactile signals. Smaller differences in optical design or manufacturing processes can create significant discrepancies in sensor output, causing machine learning models trained on a sensor to work poorly when used to others.
Computer vision models have been widely applied to vision -based tactile images because of their inherent visual nature. Researchers have adapted to representation methods from representation methods from the vision community, where contrasting learning is popular for developing tactile and visual-tactile representations for specific tasks. Auto-coding methods of representation are also being investigated where some researchers use masked auto-nodes (MAE) to learn tactile representations. Methods such as general multimodal representations use several tactile data sets in LLM frames, coding of sensor types such as tokens. Despite these efforts, current methods often require large data sets, treat sensor types such as fixed categories and lack the flexibility to generalize to unseen sensors.
Researchers from the University of Illinois Urbana-Champaign suggested Sensor-Invariant Tactile representations (SITR), a tactile representation to transfer across different vision-based tactile sensors in a zero-shot way. It is based on the assumption that achieving sensor transfer requires learning of effective sensor-invariant representations through exposure to different sensor variations. It uses three core innovations: using light-to-aquire calibration images to characterize individual sensors with a transformer codes, apply monitored contrastive learning to emphasize geometric aspects of tactile data across multiple sensors and develop a large-scale synthetic data that contains 1 m-examples across 100 Sensorchon figures.
Researchers used the tactile image and a set of calibration images for the sensor as input to the network. The sensor background is drawn from all input images to isolate the pixel color changes. After vision, these images are projected linearly into tokens, where calibration images only require tokenization once per day. Sensor. Furthermore, two supervisory signals control the education process: a pixel-venor normal card construction loss for output-patch-tokens and a contrasting loss for the class gauge. During premium, a slight decoder the contact surface reconstructs as a normal card from the code’s output. In addition, SITR uses monitored contrasting learning (SCL) that expands traditional contrastive approaches by using label information to define equality.
In object classification tests using the researchers’ data sets in the real world, SITR transforms all baseline models when transferred across different sensors. While most models are doing well in settings without transfer, they fail to generalize when tested on different sensors. It shows SITR’s ability to capture meaningful, sensor-in-varied features that remain robust despite changes in the sensor domain. In positur testing tasks, where the goal is to estimate 3-DOF position changes using initial and final tactile images, reduces the Sitr Root Mead Square error by approx. 50% compared to basic lines. Unlike classification results, image-for-training only improves marginal positions, which shows that features learned by natural images may not be effectively transferred to tactile domains for precise regression tasks.
In this document, researchers introduced Sitr, a tactile representation frame that transmits across different vision-based tactile sensors in a zero-shot way. They constructed large-scale, sensor-adapted data sets using synthetic and real world data and developed a method of training SITR to capture dense, sensor-invariant features. SITR represents a step towards a comprehensive approach to tactile sensing, where models can generalize seamlessly across different sensor types without retraining or fine tuning. This breakthrough has the potential to accelerate progress in robot manipulation and tactile research by removing a key barrier to the adoption and implementation of these promising sensor technologies.
Check out the paper and the code. All credit for this research goes to the researchers in this project. You are also welcome to follow us on Twitter And don’t forget to join our 85k+ ml subbreddit.
🔥 [Register Now] Minicon Virtual Conference On Open Source AI: Free Registration + Certificate of Participation + 3 Hours Short Event (12 April, at [Sponsored]
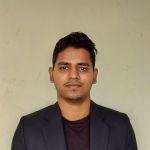
Sajjad Ansari is a last year bachelor from IIT KHARAGPUR. As a technical enthusiast, he covers the practical uses of AI focusing on understanding the impact of AI technologies and their real world. He aims to formulate complex AI concepts in a clear and accessible way.
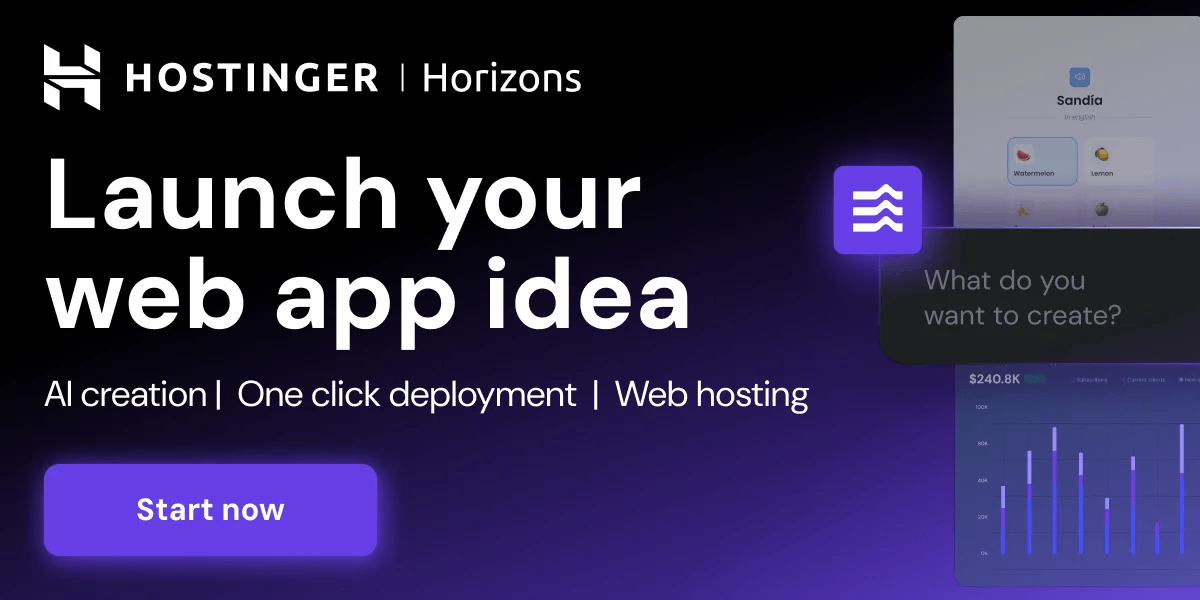