Ragrams have gained attention to their ability to improve LLMs by integrating external knowledge sources, helping to address restrictions such as hallucinations and outdated information. Traditional RAG approaches are often dependent on the surface level documents, despite their potential, lack deeply embedded insights in texts or overlook information spread over several sources. These methods are also limited in their utility, primarily catering for simple question-strain tasks and struggling with more complex applications, such as synthesis insights from various qualitative data or analyzing intricate legal or business content.
While previous RAG models improved the accuracy of tasks such as summary and QA in open domain, their retrieval mechanisms lacked depth to extract nuanced information. Newer variations, such as Iter-Retergen and Self-Scratch, try to control multi-step ranks, but are not suitable for non-composed tasks such as those examined here. Parallel efforts in insight extraction have shown that LLMs can effectively my detailed, context -specific information from unstructured text. Advanced techniques, including transformer -based models such as Openie6, have refined the ability to identify critical details. LLMs are increasingly used in Keyphrase extraction and document mineral, showing their value beyond basic collection tasks.
Researchers at Megagon Labs introduced Insightrag, a new frame that improves traditional fetch-augmented generation by incorporating an intermediate insight extraction step. Instead of relying on surface level, Insightrag First uses an LLM to identify the most important information needs for an inquiry. A domain -specific LLM retrieves relevant content in line with these insights, which generates a final, contextual response. Evaluated on two scientific paper data sets, Insight Raw surpassed significant standard rag methods, especially in tasks involving hidden or multi-source information and citation recommendation. These results highlight its broader usability in addition to standard question-strain tasks.
Insightrag includes three main components designed to tackle the shortcomings of traditional rag methods by incorporating an intermediate stage that focuses on extracting task specific insights. First, the Insight identifier analyzes the Input Question to determine its central information needs that acts as a filter to highlight relevant context. Next, Insight Miner uses a domain-adapted LLM, specifically a continuous pre-formed Llama-3.2 3B model, to retrieve detailed content in line with this insight. Finally, the response generator combines the original query with the extracted insight using another LLM to generate a contextual rich and accurate output.
To evaluate the Insight Rag, the researchers constructed three benchmarks using abstracts from the Aan and OC data sets with a focus on various challenges in recycling-augmented generation. For deeply buried insights, they identified the subject-relation object triples where the object only appears once, making it harder to detect. For insight into several source, they chose triple with several objects scattered across documents. Eventually, they assessed for non-QA assignments such as citation recommendation whether insight could guide relevant matches. Experiments showed that Insightrag consistently surpassed traditional RAG, especially when handling subtle or distributed information, with Deepseek-R1 and Llama-3.3 models showing strong results across all benchmarks.
In conclusion, Insightrag is a new frame that improves traditional cloth by adding an intermediate stage that focuses on extracting key insights. This method tackles the limitations of standard cloth, such as lack of hidden details, integration of multi-document information and handling tasks in addition to questions that answer. Insightrag first uses large language models to understand the underlying needs of an inquiry and then retrieve content in line with this insight. Evaluated on scientific data sets (Aan and OC) the consistent conventional cloth exceeded. Future instructions include expansion to fields such as law and medicine, introducing hierarchical insight extraction, handling of multimodal data, incorporating expert input and exploring transfer across domains.
Check out Paper. All credit for this research goes to the researchers in this project. You are also welcome to follow us on Twitter And don’t forget to join our 90k+ ml subbreddit.
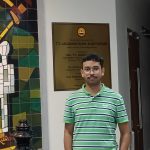
Sana Hassan, a consultant intern at MarkTechpost and dual-degree students at IIT Madras, is passionate about using technology and AI to tackle challenges in the real world. With a great interest in solving practical problems, he brings a new perspective to the intersection of AI and real solutions.
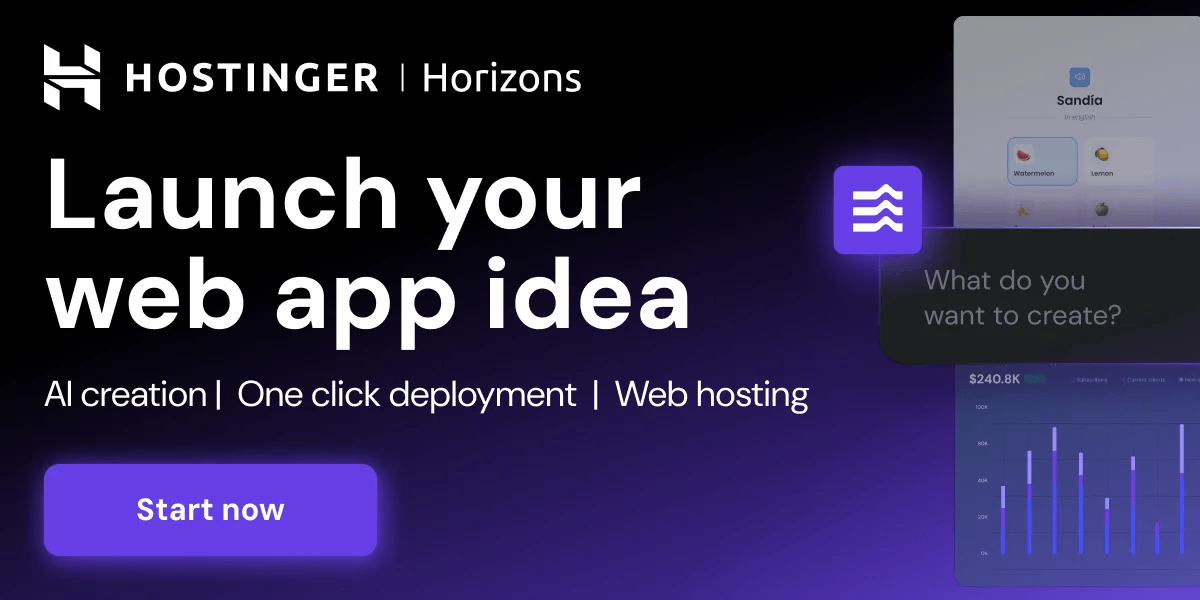