Audio Diffusion Models have achieved speech, music and foley sound synthesis of high quality, yet they are predominantly distinguished by testing rather than parameter optimization. Tasks such as physically informed impact sounding or quick-driven source separation require models that can adjust explicit, interpretable parameters under structural limitations. Performance Distribution (SDS)-which has driven text-to-3D and image editing by backing propagates through prior diffusion priority — is not yet used for audio. Adaptation of SDS to sound diffusion allows optimization of parametric sound representations without gathering large task-specific data sets that brodge singer modern generative models with parametrated synthesis work.
Classic audio techniques-such as frequency modulation (FM) synthesis that uses operator modulated oscillators to create rich Timbres and physically grounded influence sound simulators-giver compact, interpretable parameters. Similarly, source separation has evolved from matrix factorization to neural and text -controlled methods for insulating components such as vocals or instruments. By integrating SDS updates with prior audio diffusion models, you can utilize learning generative conditions to guide FM parameters optimization, impact simulators or separation masks directly from high-level prompts that unite signaling workers with flexibility in the modern diffusion-based generation.
Researchers from Nvidia and MIT introduce audio-sds, an extension of SDS to text-conditioned audio diffusion models. Audio-SDS utilizes a single preconceived model to perform different audio tasks without requiring specialized data sets. Distilling generative prior in parametric sound representations facilitates tasks such as Impact Sound simulation, FM synthesis parameter calibration and source separation. The frame combines data -driven prior with explicit parameter control and produces perceptually compelling results. Key enhancements include a stable decoder-based SDS, Multistep-Denoising and a Multiscala spectrogram method for better high-frequency details and realism.
The study discusses the use of SDS to sound diffusion models. Inspired by Dreamfusion, SDS stereo -sound generates through a rendering function, improves performance by bypassing codes and focusing instead on the decoded sound. Methodology is improved by three changes: To avoid coding instability, emphasize spectrogram functions to highlight high-frequency details and use multi-step denoising for better stability. Applications of Audio-SDs include FM synthesizers, influence of sound synthesis and source separation. These tasks show how SDS adapts to different audio domains without retraining, ensuring that synthesized audio is consistent with textual PROMPS while maintaining high faith.
The performance of the Audio-SDS frame is demonstrated across three tasks: FM synthesis, influence of synthesis and source separation. The experiments are designed to test the effectiveness of the framework using both subjective (listening test) and objective measurements such as clap scores, distance to the ground truth and signal-to-distortion conditions (SDR). Pretrained models, such as the stable audio information check, are used for these tasks. The results show significant sound synthesis and separation improvements with a clear adjustment to text recordings.
Finally, the study introduces Audio-SDS, a method that extends SDS to text-conditioned audio diffusion models. Using a single preconceived model, Audio-SDS enables a variety of tasks, such as simulation of physically informed strikes, adjusting FM synthesis parameters and performing source separation based on prompt. The procedure unites data -driven prior with custom representations, eliminating the need for large, domain -specific data sets. While there are challenges in model coverage, latent coding of artifacts and optimization sensitivity, Audio-SDS demonstrates the potential of distillation-based methods of multimodal research, especially in sound-related tasks.
Check Paper and project page. All credit for this research goes to the researchers in this project. You are also welcome to follow us on Twitter And don’t forget to join our 90k+ ml subbreddit.
Here is a brief overview of what we build on MarkTechpost:
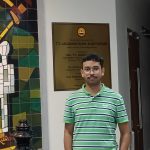
Sana Hassan, a consultant intern at MarkTechpost and dual-degree students at IIT Madras, is passionate about using technology and AI to tackle challenges in the real world. With a great interest in solving practical problems, he brings a new perspective to the intersection of AI and real solutions.