Despite the remarkable progress of large language models (LLMs), there are still critical challenges. Many models exhibit restrictions on nuanced reasoning, multilingual skills and calculation efficiency. Often models are either very skilled in complex tasks, but slow and resource -intensive or fast but prone to superficial output. Furthermore, scalability across different languages ​​and long context tasks remains a bottleneck, especially for applications that require flexible reasoning styles or longhorous memory. These questions limit the practical implementation of LLMs in dynamic environments in the real world.
Qwen3 just released: a targeted response to existing holes
Qwen3The latest release in the Qwen family of models developed by Alibaba Group aims to systematically tackle these limitations. QWEN3 introduces a new generation of models specifically optimized for hybrid reasoning, multilingual understanding and effective scaling across parameter sizes.
The QWEN3 series is expanded with the foundation laid by previous QWEN models that offer a wider portfolio of close and blend of experts (MOE) architectures. QWEN3 models designed for both research and production use cases are targeted at applications that require adaptable problem solving across natural language, coding, mathematics and broader multimodal domains.
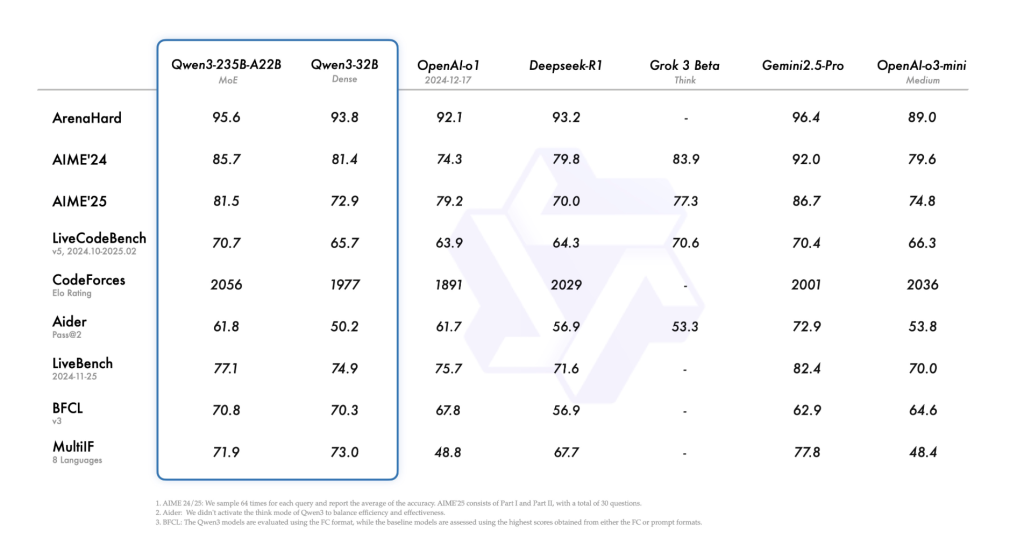
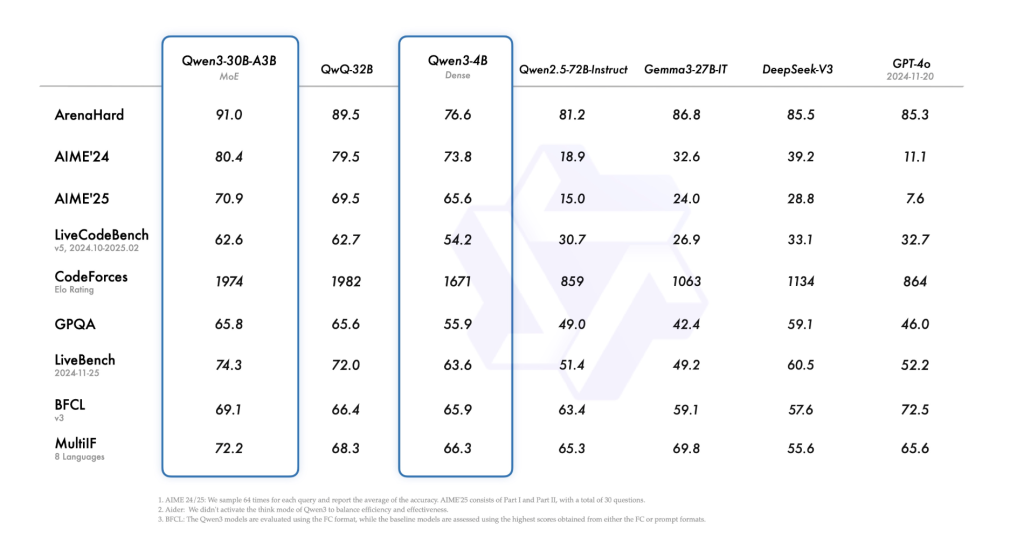
Technical innovations and architectural improvements
Qwen3 differs with several important technical innovations:
- Hybrid reasoning:
A core innovation is the model’s ability to dynamically switch between “thinking” and “non-thinking”. In “Thinking” state, QWEN3 participates in step-by-step logical reasoning decisive for tasks such as mathematical evidence, complex coding or scientific analysis. In contrast, “non -thinking” mode provides direct and effective answers to simpler queries that optimize latency without sacrificing correctness. - Extended multilingual coverage:
QWEN3 expands its multilingual capabilities significantly, supporting over 100 languages ​​and dialects, which improves accessibility and accuracy across different linguistic contexts. - Flexible model sizes and architectures:
The QWEN3 setup includes models ranging from 0.5 billion parameters (close) to 235 billion parameters (MOE). The flagship model, QWEN3-235B-A22BActivates only 22 billion parameters per year. Inference, which enables high performance while maintaining manageable calculation costs. - Long context support:
Certain Qwen3 models support context windows up to 128,000 tokensWhich improves their ability to process long documents, code bases and multi-swing conversations without degradation in performance. - Advanced training data set:
QWEN3 utilizes a refreshed, diversified corpus with improved data quality control for the purpose of minimizing hallucinations and improving generalization across domains.
In addition, the QWEN3 base models are released under an open license (subject to specified use cases), enabling the research and open source community to experiment and build on them.
Empirical results and benchmark -insight
Benchmarking results illustrate that QWEN3 models work competitively against leading contemporary:
- The QWEN3-235B-A22B Model achieves strong results across coding (Human Revel, MBPP), mathematical reasoning (GSM8K, Mathematics) and General Knowledge Benchmarks competing with Deepseek-R1 and Gemini 2.5 Pro Series models.
- The Qwen3-72B and QWEN3-72B chat Models demonstrate solid instructional and chat features that show significant improvements over the previous QWEN1.5 and the QWEN2 series.
- Especially QWEN3-30B-A3BA smaller MOE variant with 3 billion active parameters surpasses QWEN2-32B on several standard benchmarks, showing improved efficiency without a balance in accuracy.
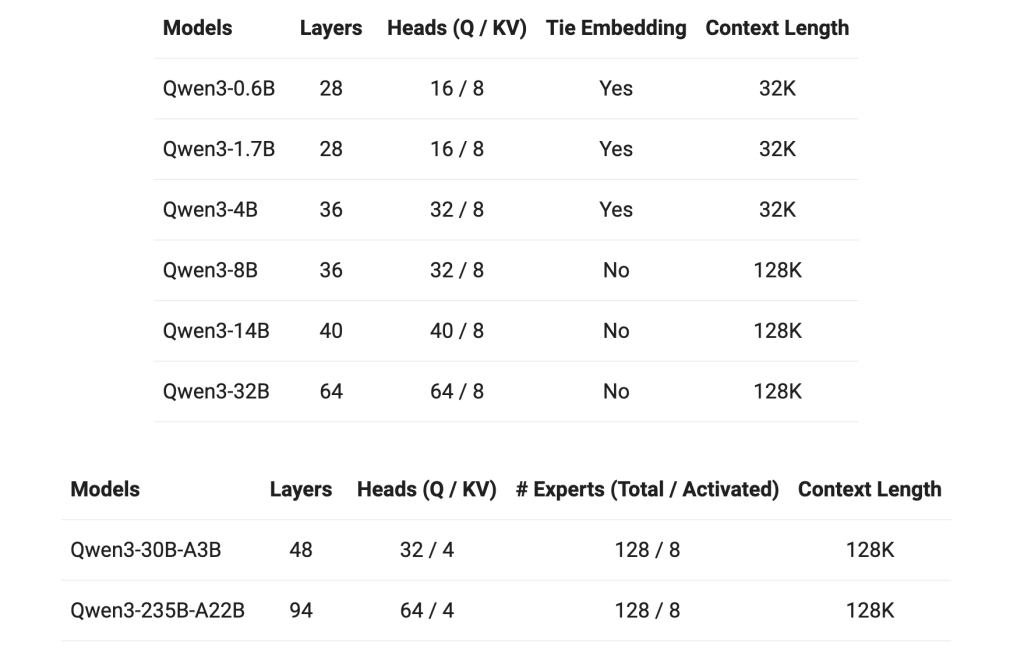
Early evaluations also indicate that QWEN3 models exhibit lower hallucination speeds and more uniform multi-turn dialogue performance compared to previous QWEN generations.
Conclusion
QWEN3 represents a thought -provoking development in great language model development. By integrating hybrid reasoning, scalable architecture, multilingual robustness and effective calculation strategies, QWEN3 addresses many of the key challenges that continue to affect LLM implementation today. Its design emphasizes adaptability – making it equally suitable for academic research, business solutions and future multimodal applications.
Instead of offering step -by -step improvements, QWEN3 redefits several important dimensions of LLM design, which sets a new reference point for balancing performance, efficiency and flexibility in increasingly complex AI systems.
Check Blog, models about embraced face and github —side. Nor do not forget to follow us on Twitter and join in our Telegram Channel and LinkedIn GrOUP. Don’t forget to take part in our 90k+ ml subbreddit.
🔥 [Register Now] Minicon Virtual Conference On Agentic AI: Free Registration + Certificate for Participation + 4 Hours Short Event (21 May, 9- 13.00 pst) + Hands on Workshop
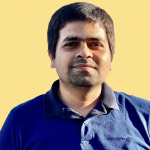
Asif Razzaq is CEO of Marketchpost Media Inc. His latest endeavor is the launch of an artificial intelligence media platform, market post that stands out for its in -depth coverage of machine learning and deep learning news that is both technically sound and easily understandable by a wide audience. The platform boasts over 2 million monthly views and illustrates its popularity among the audience.
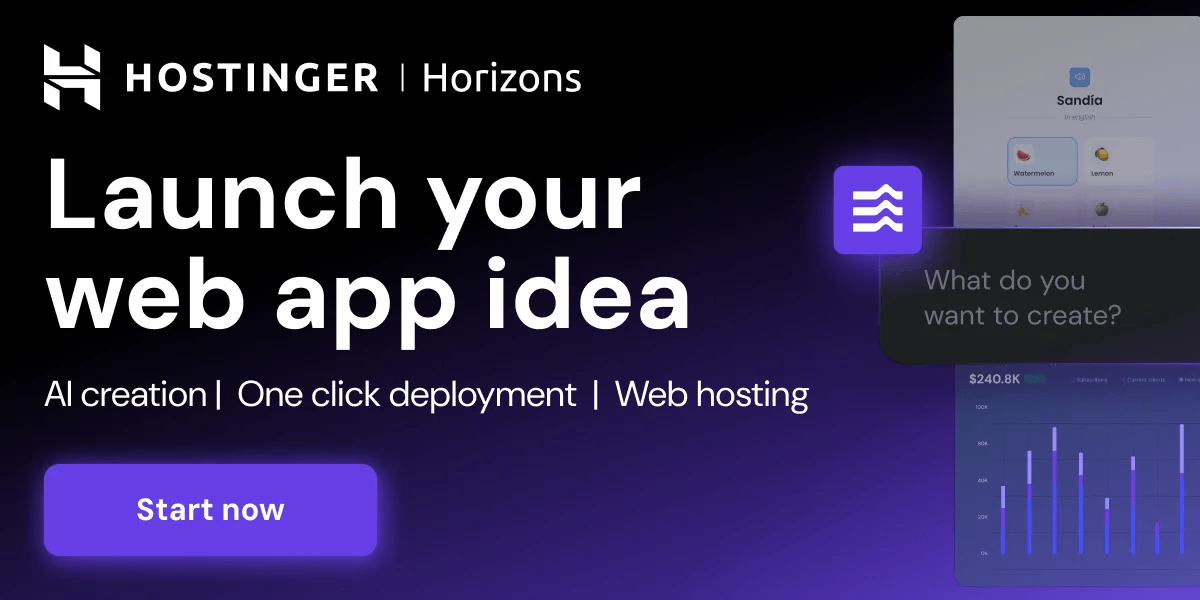