Recent progress in large language models (LLMS) has enabled the development of AI-based code products that can generate, change and understand the software code. However, the evaluation of these systems remains limited, often limited to synthetic or narrow scoped benchmarks, primarily in Python. These benchmarks rarely reflect the structural and semantic diversity of the real world code bases, and as a result, many agents monitor benchmark-specific patterns rather than demonstrating robust, transferable capabilities.
AWS introduces SWE-polybench: a more comprehensive evaluation framework
To tackle these challenges, AWS AI Labs have introduced SWE-POLYBCA multilingual benchmark at the depot level designed for execution-based evaluation of AI coding agents. Benchmark spans 21 GITHUB stocks across four widely used programming language java, JavaScript, Typescript and Python-tinging 2,110 tasks that include bug fixes, functional implementations and code factors.
Unlike previous benchmarks, SWE-polybench contains Real Pull Requests (PRS), which closes actual problems and includes associated test cases, enabling verifiable evaluation. A minor, stratified subgroup—SWE-POLYBBENCH500– has also been released to support faster experimentation while retaining the task and language diversity.
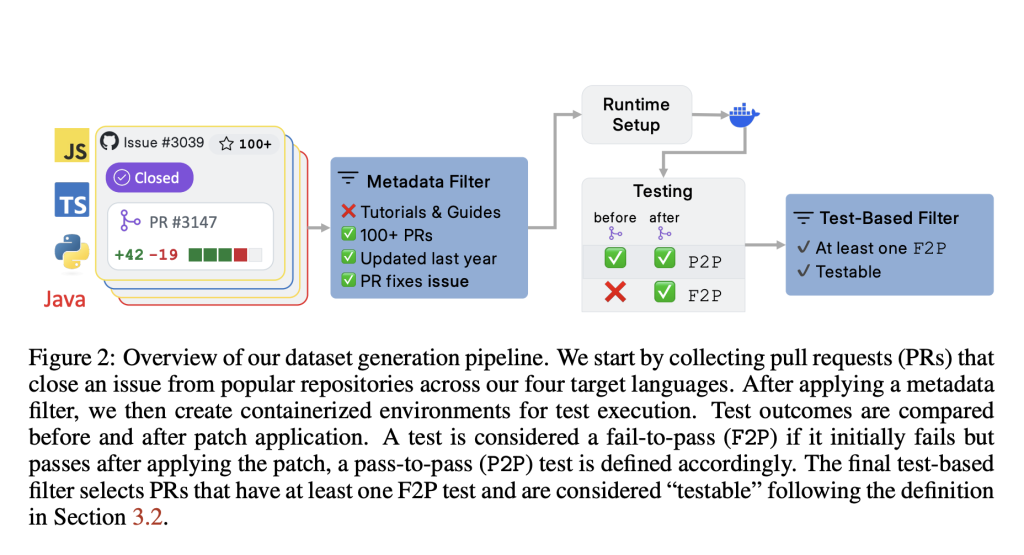
Technical structure and evaluation metrics
SWE-polybench adopts an execution-based evaluation pipeline. Each task includes a depot snapshot and a problem statement derived from a GitHub problem. The system uses the associated soil truthful in a containerized test environment that is configured for the respective language ecosystem (eg the stomach for Java, NPM for JS/TS, etc.). The benchmark then measures results using two types of unit test: Failure-to-Pass (F2P) and Pass-to-Pass (P2P).
To provide a more granular assessment of coding agents introducing SWE-polybench Concrete syntax wood (cst)– -based measurements. These include both the file level and the node level-retrieval score, assessment of the agent’s ability to locate and change relevant sections of the code base. These measurements provide insights beyond binary passes/failed results, especially for complex changes with multiple files.
Empirical evaluation and observations
Three open source coding agents-AiderAt SWE-AGENEand Agentless–Ibet adapted to Sweet-Polybench. Everyone used Anthropic’s Claude 3.5 as the underlying model and was changed to deal with the requirements for multilingual, application level on the benchmark.
The evaluation revealed remarkable differences in performance across languages ​​and types of tasks. For example, agents best priested on Python tasks (up to 24.1% pass rate), but struggled with Typescript (as low as 4.7%). Despite its higher complexity in average knot changes, Java achieved higher success rates than Typescript, suggesting that prior exposure and syntax confidentiality play a critical role in model performance.
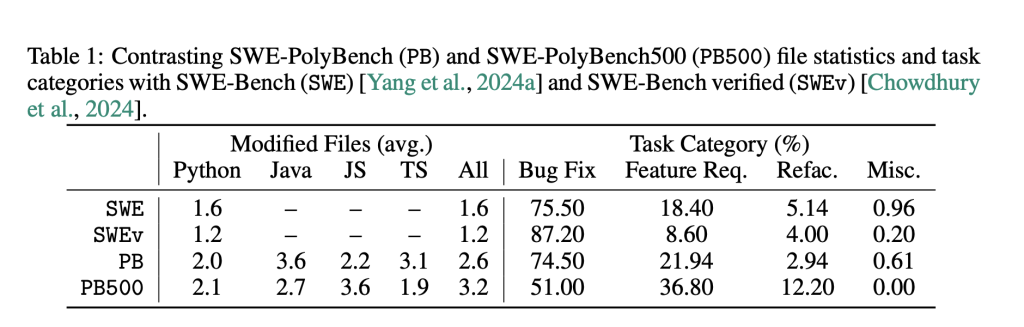
Performance also varied with task complexity. Tasks limited to single function or single class changes provided higher success rates (up to 40%), while those who required changes in mixed or multi-file saw a significant decrease. Interestingly, high -fetching precision and revocation – especially for file and CST node identification – not always for higher care speeds, indicating that code location is necessary but inadequate for problem solving.
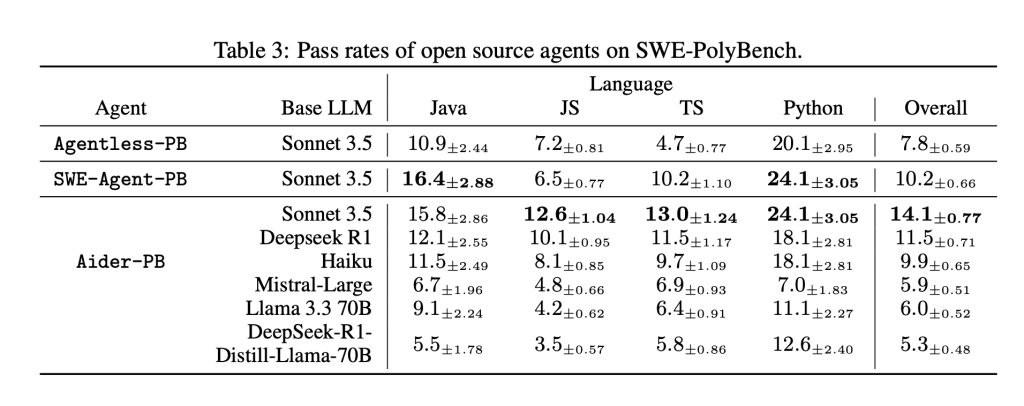
Conclusion: Against Robust Evaluation of AI coding agents
SWE-polybench presents a robust and nuanced evaluation framework for coding agents that address key restrictions in existing benchmarks. By supporting multiple programming languages, covering a wider range of task types and incorporating syntax-tuning measurements, it offers a more representative assessment of an agent’s applicability in the real world.
Benchmark reveals that although AI agents exhibit promising abilities, their performance remains inconsistent across languages ​​and tasks. SWE-polybench provides a foundation for future research aimed at improving generalizability, robustness and reasoning features of AI-coding assistants.
Check out the AWS DEVOPS blog, Hugging Face-Sweethe-Polybench and GitHub-Sweethe-Polybench. Nor do not forget to follow us on Twitter and join in our Telegram Channel and LinkedIn GrOUP. Don’t forget to take part in our 90k+ ml subbreddit.
🔥 [Register Now] Minicon Virtual Conference On Agentic AI: Free Registration + Certificate for Participation + 4 Hours Short Event (21 May, 9- 13.00 pst) + Hands on Workshop
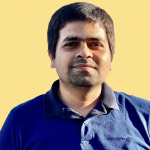
Asif Razzaq is CEO of Marketchpost Media Inc. His latest endeavor is the launch of an artificial intelligence media platform, market post that stands out for its in -depth coverage of machine learning and deep learning news that is both technically sound and easily understandable by a wide audience. The platform boasts over 2 million monthly views and illustrates its popularity among the audience.
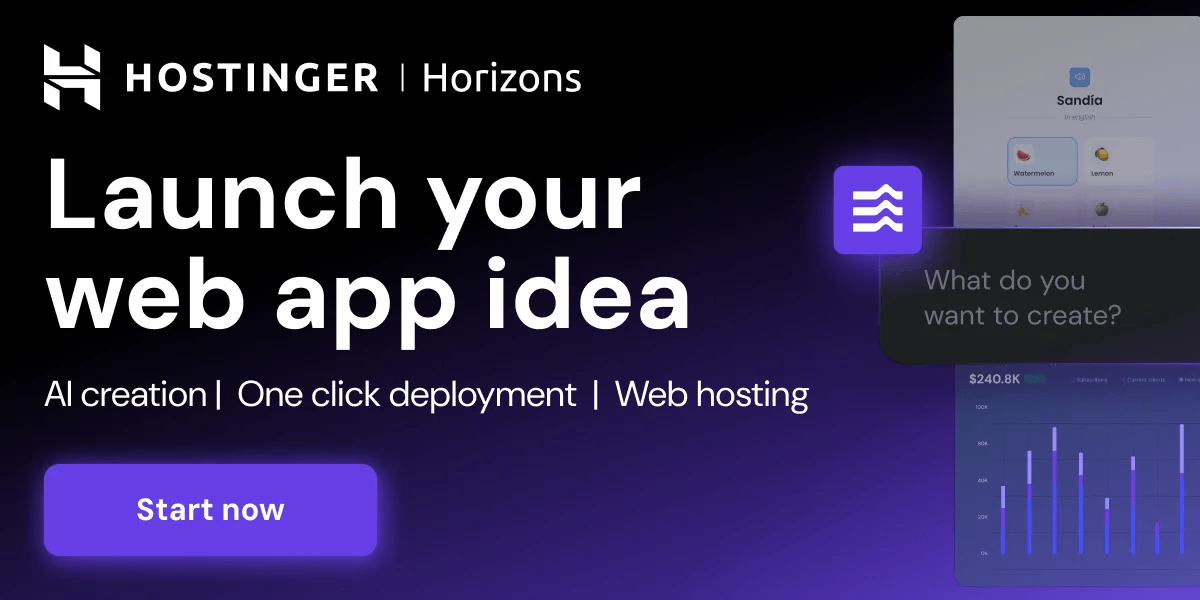