Humans have an innate ability to treat raw visual signals from the retina and develop a structured understanding of their surroundings, identify objects and movement patterns. An important goal of machine learning is to reveal the underlying principles that enable such unattended human learning. An important hypothesis, the predictable principle of functioning, suggests that representations of consecutive sensory inputs must be predictable to each other. Early methods, including slow functional analysis and spectrum techniques, aimed to maintain temporal consistency while preventing the representation of collapse. Recent approaches include Siamese networks, contrastive learning and masked modeling to ensure meaningful representation development over time. Instead of focusing solely on temporal invariance, modern techniques train predictor networks to map functional relations across different time steps, using frozen coders or training both the codes and the predictor at the same time. This predictable framework has been used successfully across modalities such as images and audio, with models such as Jepa that utilize common in -depth architectures to predict lack of information information effectively.
Progress in self-monitored learning, especially through vision transformers and joint injury architectures, has significantly improved modeling and representation learning. Spatiotemporal masking has expanded these improvements to video data and improved the quality of learned representations. In addition, cross -tested pool mechanisms have refined masked auto -coders, while methods such as Byol reduce representation collapse without relying on handmade increases. Compared to Pixel-Space Reconstruction, it makes it possible to predict in the function room models to filter irrelevant details, leading to effective, adaptable representations that generalize well across tasks. Recent research emphasizes that this strategy is computational and effective across domains such as images, audio and text. This work extends this insight into video and shows how predictable functional learning improves spatiotemporal representation quality.
Researchers from Fair at Meta, Inria, École Normal Supérieure, CNRS, PSL Research University, Univ. Gustave Eiffel, Courant Institute and New York University introduced V-JEPA, a vision model that is exclusively trained for functioning for unattended video learning. Unlike traditional approaches, V-JEPA is not dependent on prior coders, negative samples, reconstruction or text monitoring. Educated on two million public videos achieves the strong performance on movement and appearance-based tasks without fine tuning. In particular, V-JEPA surpasses other methods on something-something-V2 and remains competitive at Kinetics-400, which shows that functional prediction can only produce effective and adaptable visual representations with shorter training duration.
The methodology involves training a foundation model for object -centered learning using video data. First, a neural network draws object-centered representations from video frames that capture movement and appearance signals. These representations are then refined through contrastive learning to increase objectness. A transformer -based architecture addresses these representations to model object interactions over time. The frame is trained on a large -scale data set that optimizes for reconstruction accuracy and consistency across frames.
V-JEPA is compared to pixel prediction methods using similar model architectures and shows superior performance across video and image tasks in frozen evaluation, other than image classification. With fine tuning, the Vit-L/16-based models surpass and match Hiera-L while requiring fewer training samples. Compared to advanced models, V-JEPA stands out in motion and video tasks, training more effectively. It also demonstrates strong ethics efficiency and surpasses competitors in low -shot settings by maintaining accuracy with fewer labeled examples. These results highlight the benefits of functional prediction in learning effective video presentations with reduced calculation and data requirements.
Finally, the study examined the effectiveness of functional prediction as an independent measure of unattended video learning. It introduced V-JEPA, a set of visual models that are trained clean through self-transmitted function prediction. V-JEPA works well across different image and video tasks without requiring parameter adaptation, surpassing previous video presentation methods in frozen evaluations for action recognition, spatiotemporal action detection and image classification. Pretraining on videos improves its ability to capture fine -grained movement details where large image models are fighting. In addition, V-JEPA demonstrates strong ethics efficiency and maintains high performance, even when there are limited labeled data available for downstream tasks.
Check out the paper and blog. All credit for this research goes to the researchers in this project. You are also welcome to follow us on Twitter And don’t forget to join our 75k+ ml subbreddit.
🚨 Recommended Reading AI Research Release Nexus: An Advanced System Integrating Agent AI system and Data Processing Standards To Tackle Legal Concerns In Ai Data Set
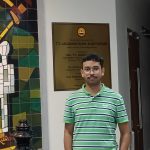
Sana Hassan, a consultant intern at MarkTechpost and dual-degree students at IIT Madras, is passionate about using technology and AI to tackle challenges in the real world. With a great interest in solving practical problems, he brings a new perspective to the intersection of AI and real solutions.
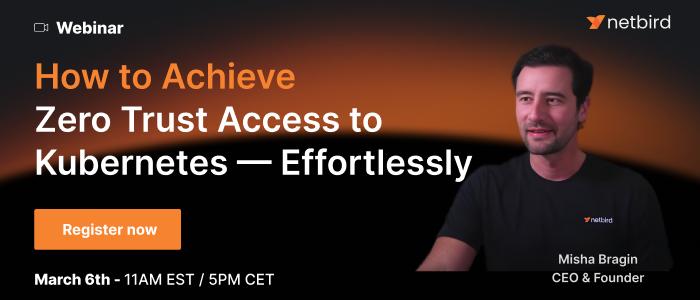