In recent years, contrastive language image models such as clips have established themselves as a standard choice for learning vision representations, especially in multimodal applications such as visual questions answers (VQA) and document understanding. These models utilize large -scale caption pair to incorporate semantic grounding via language monitoring. However, this dependence on text introduces both conceptual and practical challenges: the assumption that language is essential for multimodal performance, the complexity of acquiring adjusted data sets and the scalability limits imposed by data availability. In contrast, Visual Self-Supervised Learning (SSL)-which works without language-historically demonstrated competitive results about classification and segmentation tasks that have nevertheless been under-utilized to multimodal reasoning due to performance holes, especially in OCR and short-based tasks.
Meta releases websl models on embraced face (300m -7b -parameters)
In order to explore the capabilities of language -free visual learning in scale has meta released Web-SSL Family of Dino and Vision Transformer (Vit) modelsranging from 300 million to 7 billion parameters, now publicly available via hugging surface. These models are trained exclusively on the photo padding of Metaclip Dataset (MC-2B)—A Web scale data set consisting of two billion images. This controlled setup enables a direct comparison between websl and clip, both trained in identical data that isolates the effect of language monitoring.
The goal is not to replace clips, but to evaluate how far purely visual self-oversee can go when model and data ala no longer limit factors. This release represents a significant step towards understanding whether language monitoring is necessary or simply advantageous-to-training of high capacity vision coders.
Technical architecture and training methodology
WebSL includes two visual SSL paradigms: joint learning of learning (via Dinov2) and masked modeling (via MAE). Each model follows a standardized training protocol using 224 × 224 resolution images and maintains a frozen vision codes under downstream evaluation to ensure that observed differences can only be attributed to pretraining.
Models are trained across five capacity levels (vit-1b for vit-7b) by using only unmarked image data from MC-2B. Evaluation is performed using Cambrian-1A comprehensive 16-task VQA-Benchmark suite, which includes general vision understanding, knowledge-based reasoning, OCR and short-based interpretation.
In addition, the models are naturally supported in embracing face transformers
Library that provides available control points and trouble -free integration into research work.
Achievement insight and scaling behavior
Experimental results reveal several key findings:
- Scaling model size: WebSL models demonstrate almost log-linear improvements in VQA performance with increasing parameter numbers. In contrast, the clip’s performance plateauses are beyond 3b parameters. WebSL maintains competitive results across all VQA categories and shows pronounced gains in vision-centric and OCR & chart assignments on larger scales.
- Data composition matters: By filtering the training data to include only 1.3% of text-rich images, websl clips surpass OCR & CHART-tasks obtained up to +13.6% Gains in Ocrbench and Chartqa. This suggests it the presence of visual text aloneNot language labels, significantly improves task specific performance.
- Exercise in high resolution: Websl models Finely tuned at 518PX resolution closes additional benefit gap with high resolution models such as Siglip, especially for document-heavy tasks.
- Llm -adjustment: Without any language monitoring, WebSL shows improved adaptation to prior language models (eg Llama-3) when exposure to model size and training exposure increases. This new behavior implies that larger visual models implicitly learn functions that correlate well with textual semantics.
It is important that WebSL maintains strong performance on traditional benchmarks (image-1K-classification, ADE20K segmentation, NyUV2 depth estimation) and often surpass Metaclip and even Dinov2 under equivalent settings.
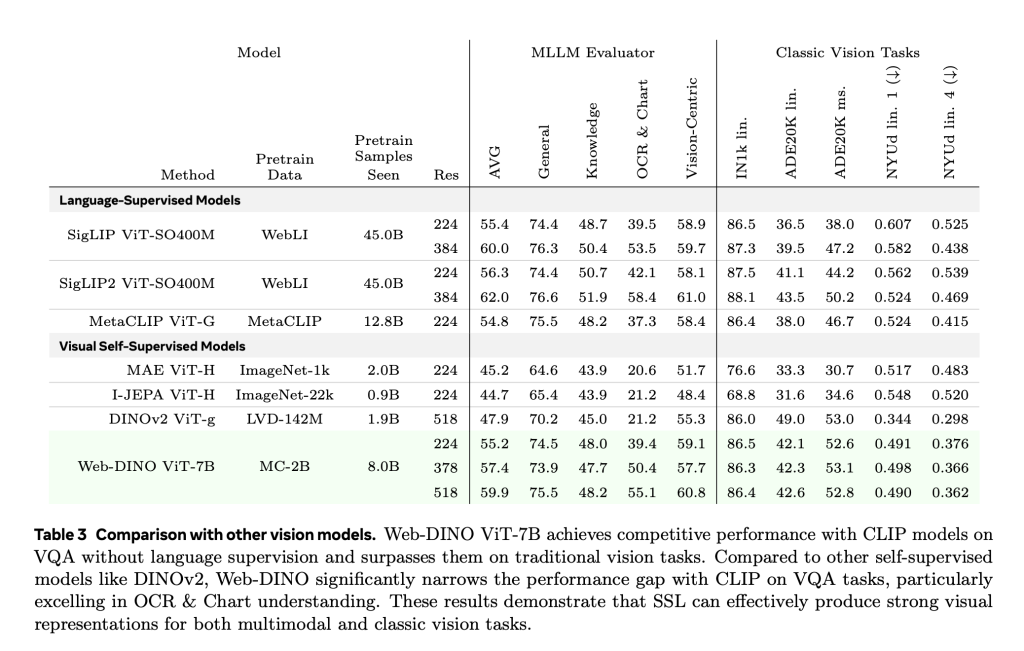
Final observations
Meta’s Web-SSL study provides strong proof that Visual Self -Trusted Learning When Siminely Soning. These findings challenge the prevailing assumption that language supervision is important for multimodal understanding. Instead, they highlight the importance of data set composition, model scale and careful evaluation across different benchmarks.
The release of models ranging from 300 m to 7b parameters enables wider research and downstream experimentation without the limitations of paired data or proprietary pipelines. As open source foundations for future multimodal systems, webSl models represent a meaningful progress in scalable, language-free vision learning.
Check Models about hug faceAt GitHub Page and Paper. Nor do not forget to follow us on Twitter and join in our Telegram Channel and LinkedIn GrOUP. Don’t forget to take part in our 90k+ ml subbreddit.
🔥 [Register Now] Minicon Virtual Conference On Agentic AI: Free Registration + Certificate for Participation + 4 Hours Short Event (21 May, 9- 13.00 pst) + Hands on Workshop
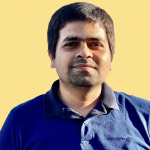
Asif Razzaq is CEO of Marketchpost Media Inc. His latest endeavor is the launch of an artificial intelligence media platform, market post that stands out for its in -depth coverage of machine learning and deep learning news that is both technically sound and easily understandable by a wide audience. The platform boasts over 2 million monthly views and illustrates its popularity among the audience.
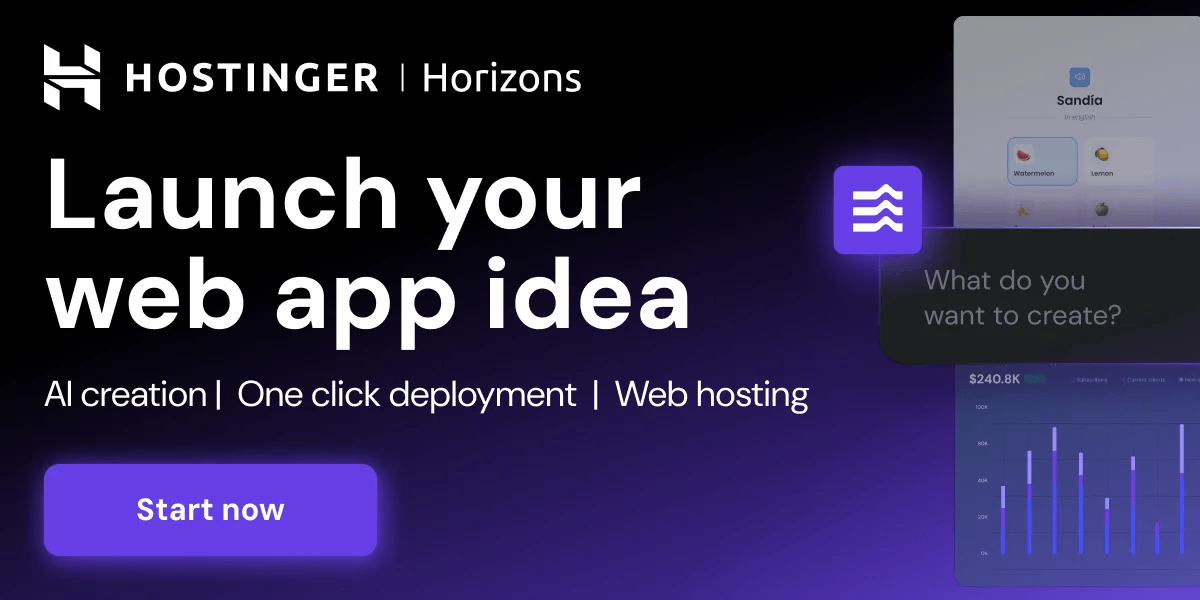