The rapid progress of large language models (LLMS) has improved their ability to generate long -shaped reactions. However, evaluation of these answers remains effectively and fairly a critical challenge. Traditionally, human evaluation has been the gold standard, but it is expensive, time -consuming and prone to bias. To mitigate these limitations, LLM-as-A-Judge paradigm has emerged and utilizes LLMs to act as evaluators. Despite this progress, LLM-AS-A-Judge models face two significant challenges: (1) Lack of human-annoted chain-of-tank (COT) rations that are important for structured and transparent evaluation, and (2) Existing approaches that depend on rigid, hand -designed evaluation components, making them difficult to generalize across different tasks and domains. These restrictions limit the accuracy and robustness of AI-based evaluation models. To overcome these problems, Meta AI has introduced EvalDplanner, a new approach designed to improve reasoning and decision making for LLM-based judges through an optimized planning equipment strategy.
Evaluation is a preference optimization algorithm specifically designed for Thinking-llm-as-a-judge Models. EvalPlanner differentiates by applying a three-step evaluation process: (1) Generating an unlimited evaluation plan, (2) implementation of the plan and (3) final judgment. Unlike previous methods, the evalcan plan does not limit reasoning traces to predefined sections or criteria. Instead, it generates flexible evaluation plans that adapt to different domains and task requirements. The system works in a self -training loop, iterative refining evaluation plans and execution strategies using Synthetically generated preference pair. By continuously optimizing oneself ensures evalnplanner More reliable, transparent and scalable evaluations Compared to existing LLM-as-A-Judge models.
The innovation behind evalplanner lies in its Structured reasoningthat separates the planning phase from the execution phase. In the planning phase, the model formulates a detailed evaluation card tailored to the specific instruction. During the execution, the model follows the step -by -step plan to assess and compare answers systematically. This two-stage separation enables better adjustment between evaluation goals and reasoning processes, leading to more accurate and explainable assessments.
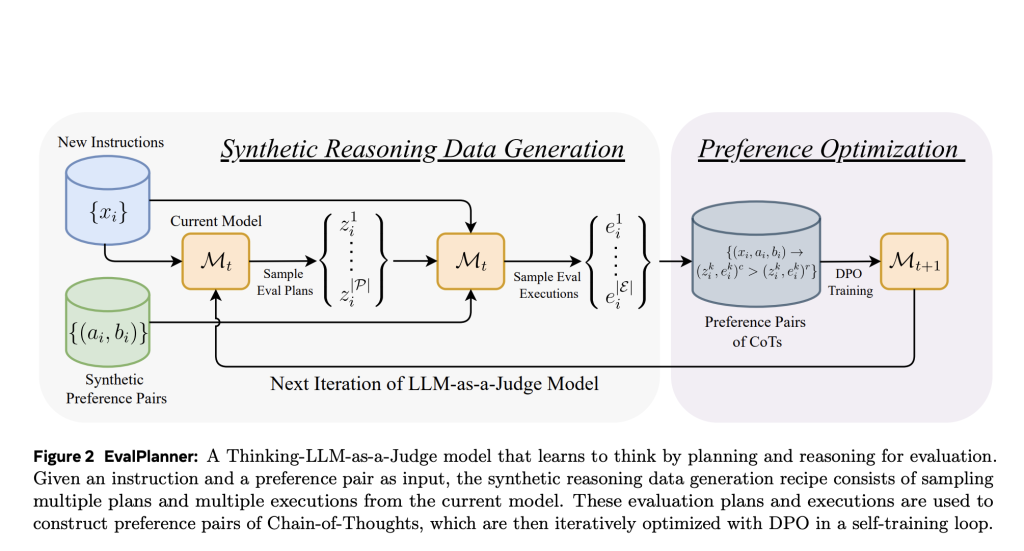
Technical details and benefits of evalnplanner
Evalplanner introduces one Self -training mechanism It continuously improves both the planning and execution components of the evaluation process. The model is utilizing Direct Preference Optimization (DPO) To iteratively improve its assessments by learning from synthetic preference pairs. These preference pairs are derived from sampling several evaluation plans and executions, allowing evaluation to identify the most effective reasoning patterns.
The primary benefits of evalcan plans include:
- Increased accuracy: By generating Unlimited evaluation plansEvaluating evaluation significantly bias and improves the judgment consistency across different tasks.
- Scalability: In contrast to manually designed evaluation heading, evaluation adapted automatically for new evaluation tasks, making it a very scalable solution.
- Efficiency: Evaltplanner achieves Advanced (Sota) Performance On different benchmarks with Fewer training examplesThere is only dependent on synthetic preference pairs rather than extensive human comments.
- Transparency: By explicitly separating the planning from execution improves evaluation Interpretability of its reasoning process, making it easier to analyze and debug.
Experimental results and performance insights
META AI evaluated evaluation across multiple reward modeling benchmarks, including Reward Bench, RM-Bench, Judgebench and Followbappestal. The results demonstrate Evalplanner’s overall performance in Evaluation of complex restrictions on multiple levels and improving existing models on various domains, such as chat -based interactions, security evaluation, coding and mathematical reasoning.
- Advanced results on Reward Bench: Evaluation obtained a score of 93.9better than leading models that are dependent on 30 times more Human-Annoted Data. This highlights the effectiveness of Evalplanner’s synthetic data -driven training methodology.
- Improved robustness on RM-bench: Evaltplanner demonstrated 8% higher accuracy Compared to previous SOTA models in handling nuanced evaluation criteria showing its ability to resist subtle bias and variations As a response quality.
- Handling superior restriction in Followbench eval: For evaluation at multiple levels of restrictions, evaluation exceeded competitive basic lines by 13%underline its ability to effectively Plan and reason through complex prompts.
- Generalization to Judge Bench: Evalplanner demonstrated strong generalization functions, achieve comparable performance with larger models Trained on extensive human-annoted data sets while using significantly fewer preference pairs.
In addition, ablation studies confirmed that Iterative optimization of evaluation plans improves performance significantly. When trained with as few as 5K synthetic preference pairEvaluated maintained competitive results and demonstrated its Data Efficiency Compared to traditional models.
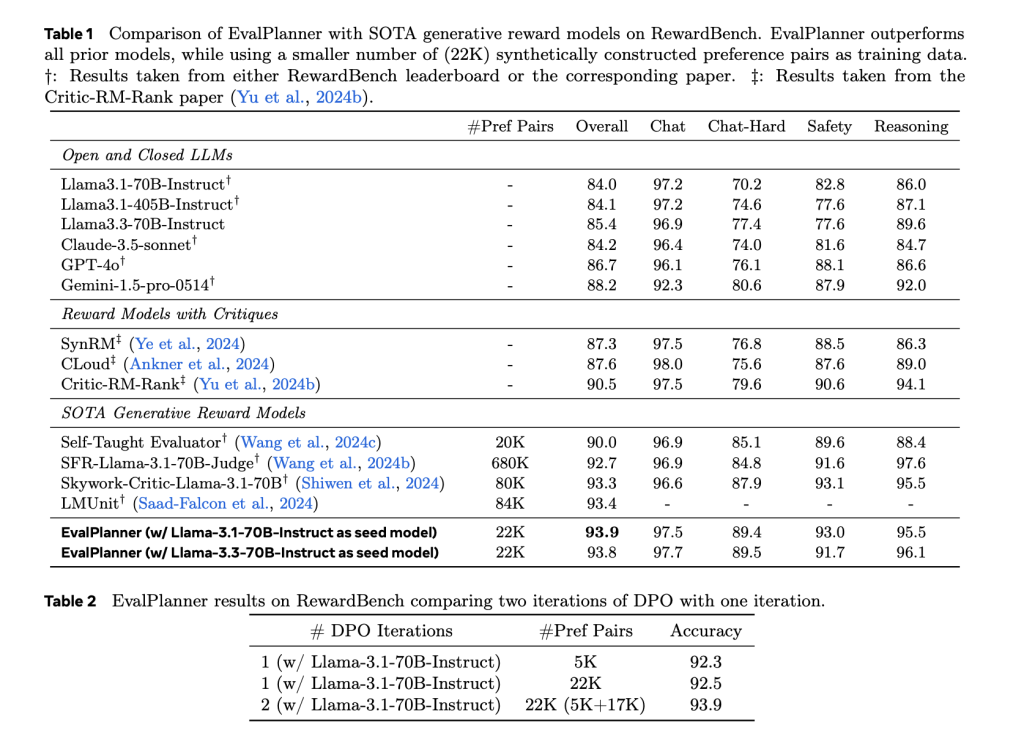
Conclusion: The future of AI-based evaluation
Evalplanner represents one Larger breakthrough In the development of AI-based evaluation frames. By combining Preference optimization, structured planning and self -trainingIt effectively addresses the limitations of existing LLM-AS-A-Judge models. Its scalability, accuracy and transparency Makes it a promising tool for automated, objective and effective Evaluation of AI-Generated Responses across different applications. As AI models continue to evolve, the Evalganner paves the way for More reliable and interpretable evaluation systemsIn the end Improving trust and justice in AI-driven decision making. Future research can explore Extending Evalplanner’s ability to reward modeling in reinforcement learning with human feedback (RLHF) pipelines and integrate it into the real world AI audit framework.
With EvalPlanner, Meta AI has set a new standard within AI evaluation, which shows that Teaching in AI to plan and resonate can improve judgment quality significantly. This progress is a crucial step toward autonomous and scalable AI managementwhich ensures that future AI systems work with larger precision, justice and accountability.
Check out the paper. All credit for this research goes to the researchers in this project. Nor do not forget to follow us on Twitter and join in our Telegram Channel and LinkedIn GrOUP. Don’t forget to take part in our 70k+ ml subbreddit.
Meet Intellagent: An Open Source Multi-Agent framework to evaluate complex conversation AI system (Promoted)
The Post Meta AI suggests evalcanes: a preference stimization algorithm for thinking-llm-as-a-judge appeared first on market post.