Multimodal AI develops rapidly to create systems that can understand, generate and respond using multiple data types within a single conversation or task, such as text, images and even video or audio. These systems are expected to operate across different interaction formats, enabling more trouble-free human-ai communication. With users who are increasingly engaging AI for tasks such as caption, text -based photo editing and style transfers, it has become important for these models to treat input and interact across modalities in real time. The boundary of the research within this domain is focused on merging capabilities when handled by separate models in total systems that can execute fluently and accurately.
An important obstacle in this area stems from incorrect adjustment between language -based semantic understanding and the visual belief required in image synthesis or editing. When separate models handle different modalities, output often becomes inconsistent, leading to poor coherence or inaccuracies in tasks that require interpretation and generation. The visual model may be distinguished in the reproduction of an image, but fails to understand the nuanced instructions behind it. In contrast, the language model can understand the prompt, but cannot shape it visually. There is also a scalability problem when models are trained in isolation; This approach requires significant calculation resources and retraining efforts for each domain. Inability to seamlessly connect vision and language to a coherent and interactive experience is still one of the fundamental problems of promoting intelligent systems.
In recent attempts to bridge this gap, scientists have combined architectures with regular visual codes and separate decoders that work through diffusion -based techniques. Tools such as tokenflow and Janus integrate token -based language models with image generation backends, but they typically emphasize pixel accuracy over semantic depth. These approaches can produce visually rich content, yet they often miss out on the contextual shades of user input. Others, like GPT-4O, have moved toward natural image generation features, but still work with limitations in deeply integrated understanding. The friction lies in translating abstract text asking for meaningful and context -conscious visuals into a fluid interaction without dividing the pipeline into incoherent parts.
Researchers from Inclusion AI, Ant Group introduced Ming-Lite-Uni, an open source frame designed to unite text and vision through an authentic multimodal structure. The system has a native Authorgressive model built on top of a fixed large language model and a fine -tuned diffusion image generator. This design is based on two core frames: Metaqueries and M2 Omni. Ming-Lite-Uni introduces an innovative component of multi-scale that can be learned tokens that act as interpretable visual devices, and a similar Multi-scale adjustment strategy to maintain the relationship between different image scales. The researchers provided all model weights and implementation openly to support social research, placement of Ming-Lite-Uni as a prototype moving towards general artificial intelligence.
The core mechanism behind the model involves the compression of visual input in structured token sequences across multiple scales, such as 4 × 4, 8 × 8 and 16 × 16 image patches, each representing different detail levels, from layout to textures. These tokens are treated with text tokenes using a large authentic transformer. Each resolution level is marked with unique starting and end tokens and assigned custom position codes. The model uses a multi-scale representation adjustment strategy that adjusts medium and output functions through an average square fault loss, ensuring consistency across layers. This technique increases the image structure quality by over 2 dB in PSNR and improves generational evaluation (Genval) scores by 1.5%. Unlike other systems that retrain all components, the Ming-Lite-uni language model holds the language model frozen and only fine-tunes the image generator, allowing faster updates and more efficient scaling.
The system was tested on various multimodal tasks, including generating text-to-image, style transfer and detailed image editing using instructions such as “Get the sheep to wear small sunglasses” or “remove two of the flowers in the image.” The model handled these tasks with high faith and contextual relocation. It maintained a strong visual quality even when it got abstract or stylistic requests such as “Hayao Miyazaki’s style” or “adorable 3D.” The training set spans 2.25 billion samples combining Laion-5B (1.55B), COYO (62 m) and zero (151 m), supplemented with filtered samples from Midjourney (5.4 m), Wukong (35 m) and other web sources (441 m). In addition, the fine-grained data set for aesthetic assessment, including AVA (255K samples), tad66k (66K), AESMMIT (21.9K) and APDD (10K), which improved the model’s ability to generally appeal to the output according to human aesthetic standards.
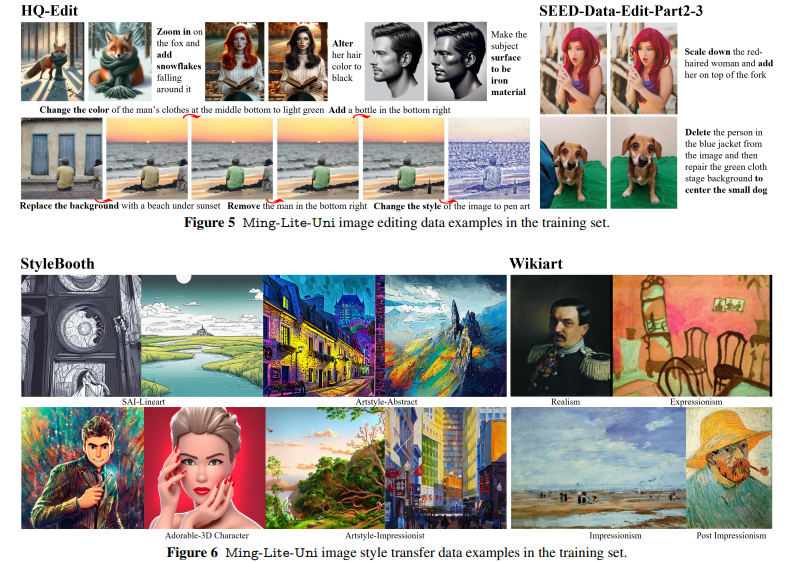
The model combines semantic robustness with high resolution image generation in a single passport. It achieves this by adjusting image and text representations at token levels across scales instead of depending on a fixed codes coding. The procedure allows autorous models to perform complex editing tasks with contextual guidance that were previously difficult to achieve. Flowmatching losses and scale-specific border markers support better interaction between the transformer and the diffusion layers. In general, the model hits a rare balance between language understanding and visual output, which places it as a significant step towards practical multimodal AI systems.
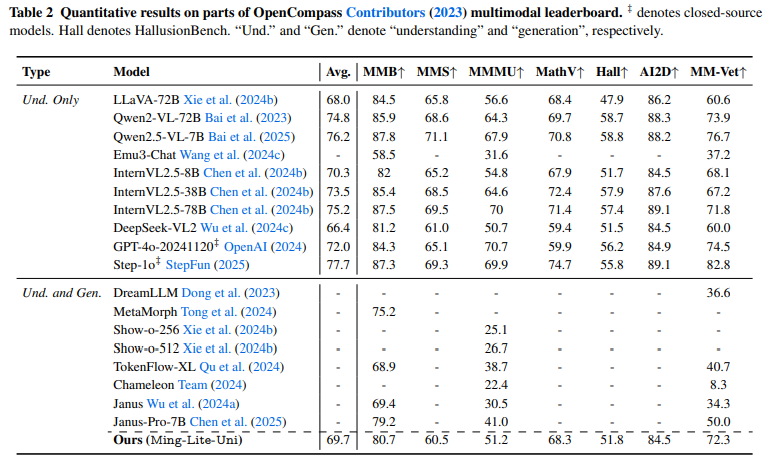
Several important takeaways from the research on Ming-Lite-Uni:
- Ming-Lite-Uni introduced a unified architecture to vision and language tasks using autorgressive modeling.
- Visual input is coded using lively tokens with multiple scales (4 × 4, 8 × 8, 16 × 16 solutions).
- The system maintains a frozen language model and trains a separate diffusion -based image generator.
- A multi-scale representation adjustment improves the context, giving an over 2 dB improvement in PSNR and a 1.5% boost in Genval.
- Educational data includes over 2.25 billion samples from public and curated sources.
- Tasks that are handled include the generation of text-to-image, image editing and visual Q&A, all treated with strong contextual relocation.
- The integration of aesthetic scoring data helps to generate visually comfortable results that are consistent with human preferences.
- Model weights and implementation are open sourced, which encourages the replication and expansion of society.
Check PaperModel about the embrace of face and github side. Nor do not forget to follow us on Twitter.
Here is a brief overview of what we build on MarkTechpost:
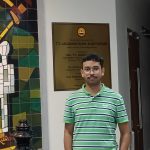
Sana Hassan, a consultant intern at MarkTechpost and dual-degree students at IIT Madras, is passionate about using technology and AI to tackle challenges in the real world. With a great interest in solving practical problems, he brings a new perspective to the intersection of AI and real solutions.