Large languages MDOELS LLMS has shown a remarkable benefit across different text and multimodal tasks. However, many applications, such as document and video understanding, requires learning in context and inference-time scaling, the ability to process and resonate over long sequences of tokens. The limited context window of LLMS poers a significant challenge in these situations, as critical information spread over long documents can be overlooked. Models often miss important information when dealing with comprehensive documents or videos that fall outside their fast-context windows. This limitation creates a need for models that can effectively handle ultra-long contexts without sacrificing performance on standard tasks.
Existing contextual expansion strategies for long context language models fall into three categories: accurate attention methods, approximate attention methods and approaches containing additional modules. Methods such as position internal polishing, NTK-attention, dynamic NTK, yarn and Clex enhance the attention mechanisms through redesigned position inputs. Recent progress includes models such as GPT-4O, Gemini and Claude, which supports extensive context windows on hundreds of thousands of tokens, but their natural nature limits reproducibility. Open source efforts as an extension of NTK-noticing scaling, but requires expensive calculation, while gradient still uses prior to contain the standard task performance.
Researchers from UIUC and NVIDIA have suggested an effective training recipe to build Ultra-Lang context LLMS from adjusted directed models pushing the limits of context lengths from 128k to 1m, 2m and 4m tokens. The method uses effective, continued prior strategies to expand the context window while using instructional optimity to maintain instructional and reasoning skills. In addition, their Ultralong-8B model achieves advanced performance across different long contracts Benchmarks. Models trained with this approach maintain competitive results on standard benchmarks that show balanced improvements in long and short context tasks. The research provides an in -depth analysis of choices of key designs that highlight the effects of scaling strategies and data composition.
The proposed method consists of two key phases: Continued prior and instructional mood. Together, these phases enable effective treatment of ultra-long input while maintaining strong performance across tasks. A yarn-based scaling method is adopted for context expansion with solid hyperparameters such as α = 1 and β = 4 rather than NTK-Aware scaling strategies. The scale factors are calculated based on target context length and use larger scaling factors for rope holes to accommodate extended sequences and reduce benefit degradation in maximum lengths. Researchers subgroups SFT dataset of high quality of high quality spans general, math and code domain for training data and further GPT-4O and GPT-4o-mini are used to refine answers and perform strict data daming.
The suggested models show superior retrieval of long context functions in the needle in a Haystack Passkey Henting Test. Baseline models such as Llama-3-8B-Instrum-Gradient-1048K consist the test, but Llama3.1-8B-Instrum and Llama-3-8B-Prolong-512K institution shows errors. In contrast, the Ultralong models achieve 100% accuracy across all input lengths and depths, showing strong retrieval. Ultralong achieves the highest average scores on the ruler of input up to 512K and 1M tokens, the highest F1 scores on LV-Val within 128k and 256k token lengths and the best performance at Infinitebench. In addition, the models maintain strong performance across general, math and code domain with average scores of 62.47, 61.06 and 60.95, exceeding the basic model’s 61.45.
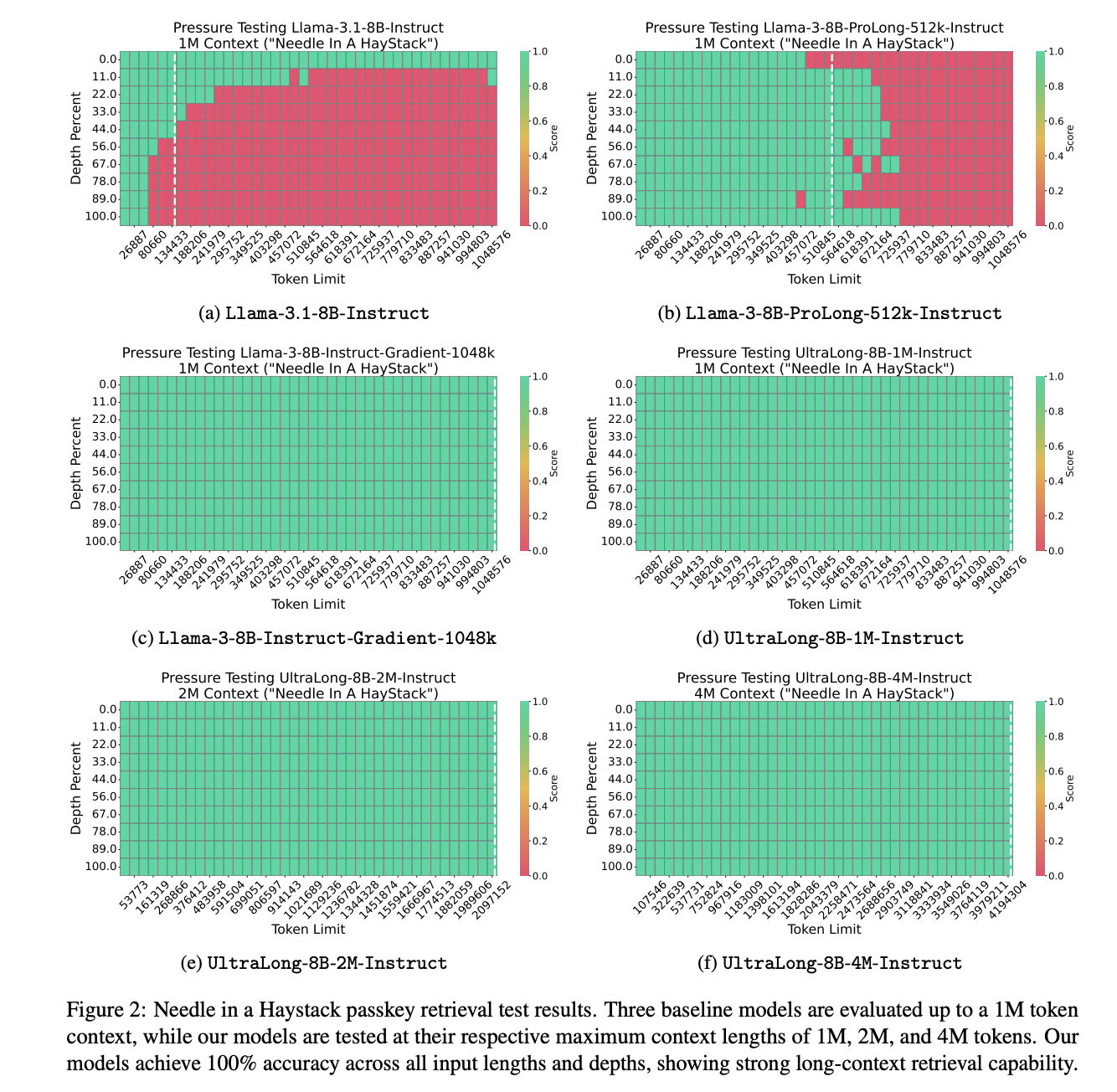
This research article introduces an effective and systematic training recipe for ultra-long context language models that extend context windows to 1m, 2m and 4m-tokens, while maintaining competitive results on standard benchmarks. The procedure combines effective continued pre -determination with instructional setting to improve long context understanding and instructional capabilities. However, this approach focuses only on SFT on instructional data sets in the instructional mood without exploring reinforcement or preference optimization. It also does not concern security adjustment. Future research includes integration of security adjustment mechanisms and exploring advanced setting strategies, further improvement of performance and reliability.
Check out Paper and model on embraced face. All credit for this research goes to the researchers in this project. You are also welcome to follow us on Twitter And don’t forget to join our 85k+ ml subbreddit.
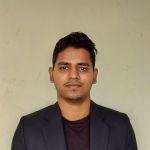
Sajjad Ansari is a last year bachelor from IIT KHARAGPUR. As a technical enthusiast, he covers the practical uses of AI focusing on understanding the impact of AI technologies and their real world. He aims to formulate complex AI concepts in a clear and accessible way.
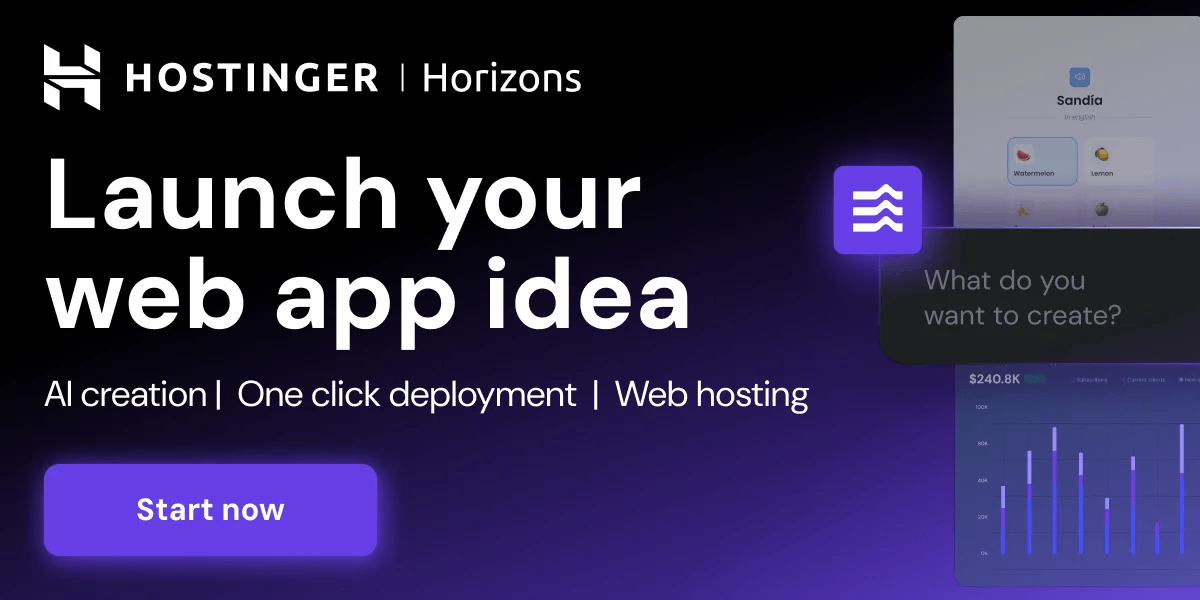