Data buttonness in generative modeling
Generative models are traditionally dependent on high quality datasets to produce samples that repeat the underlying data distribution. However, in fields such as molecular modeling or physics -based inference, the acquisition of such data may be calculated impossible or even impossible. Instead of labeled data, only a scale reward – typically derived from a complex energy function – is available to judge the quality of generated samples. This poses a significant challenge: How can you train generative models effectively without direct supervision from data?
Meta AI introduces adjoining sampling, a new learning algorithm based on scalar rewards
META AI Tackles this challenge with Adjacent samplingA new learning algorithm designed for training generative models by using only scaly reward signals. Built on the theoretical framework for stochastic optimal control (SOC), updated adjacent sampling of the training process as an optimization task over a controlled diffusion process. Unlike standard generative models, it does not require explicit data. Instead, it learns to generate high quality samples by iteratively refining them by means of a reward functional derived from physical or chemical energy models.
Ajoint -Sampling is distinguished in scenarios where only an abnormalized energy function is available. It produces samples that comply with the target distribution defined by this energy by bypassing the need for corrective methods such as sampling or MCMC which is calculated intensive.
Technical details
The basis for adjacent sampling is a stochastic differential equation (SDE) that models how sample courts develop. The algorithm learns a control operation u (x, t) u (x, t) u (x, t) so that the final state of these trajectories approaches a desired distribution (eg Boltzmann). An important innovation is its use of Mutual adjoint matching (RAM)—A Tab function that enables gradient -based updates by using only the initial and final conditions of sample courts. This side has the need to back propagat throughout the diffusion path, which greatly improves calculation efficiency.
By sampling from a known basic process and conditioning on terminal states, adjacent sampling constructs a repetition buffer of samples and gradients, allowing more optimization steps per day. Sample. This on-policy training method provides scalability unmatched by previous approaches, making it suitable for high-dimensional problems such as molecular conformer generation.
In addition, the adjoint -sampling geometric symmetries and periodic border conditions support, which allows models to respect molecular invarians such as rotation, translation and torsion. These features are crucial to physically meaningful generative tasks in chemistry and physics.
Performance Insights and Benchmark Results
Ajoint testing achieves advanced results in both synthetic and real world tasks. On synthetic benchmarks such as double well (DW-4), Lennard-Jones (LJ-13 and LJ-55) potentials, it surpasses essential base lines such as DDS and PIs, especially in energy efficiency. For example, where DDS and PIs require 1000 evaluations per year. Gradient update, uses adjacent sampling only three, with similar or better performance at Wasserstein distance and effective sample size (ASS).
In a practical setting, the algorithm was evaluated on a large-scale molecular conformer generation using the ESEN-Energ model trained on the spice-mace-off data set. Ajoint sampling, especially its Cartesian variant with prior, obtained up to 96.4% recall and 0.60 Å average RMSD, which exceeds RDKIT ENKDG-a widely used chemistry-based baseline-on background of all measurements. The method is well generalized to the Geom-Drugs data set, which shows significant improvements in revocation while maintaining competitive precision.
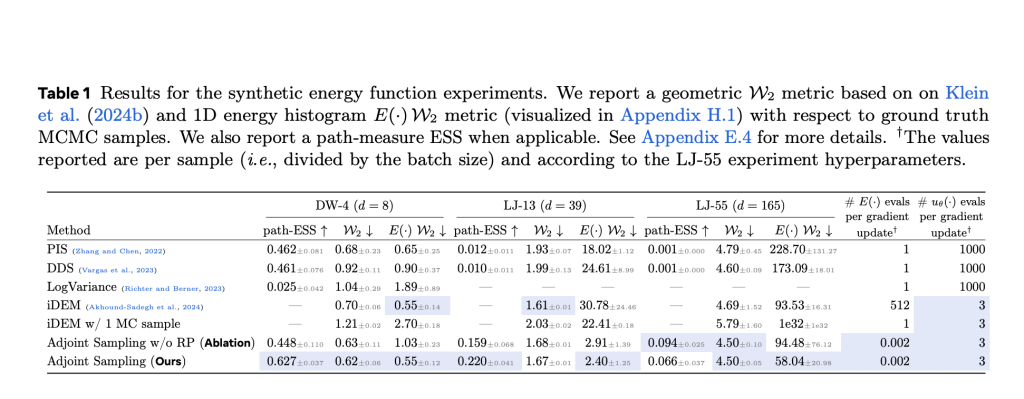
The ability of the algorithm to explore the configuration space broadly, helped by its stochastic initialization and reward-based learning, results in greater conformity diversity-critical for drug discovery and molecular design.
Conclusion: A scalable path forward for reward -driven generative models
Ajoint testing represents a big step forward in generative modeling without data. By utilizing Scalar reward signals and an effective training method that is grounded in stoccastic control, it enables scalable training of diffusion-based samplers with minimal energy evaluations. Its integration of geometric symmetries and its ability to generalize across different molecular structures place it as a basic tool in calculation chemistry and beyond.
Check the paper, the model about embraced face and github —side. All credit for this research goes to the researchers in this project. You are also welcome to follow us on Twitter And don’t forget to join our 95k+ ml subbreddit and subscribe to Our newsletter.
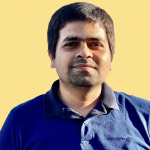
Asif Razzaq is CEO of Marketchpost Media Inc. His latest endeavor is the launch of an artificial intelligence media platform, market post that stands out for its in -depth coverage of machine learning and deep learning news that is both technically sound and easily understandable by a wide audience. The platform boasts over 2 million monthly views and illustrates its popularity among the audience.
🚨 Build Genai you can trust. ⭐ Parlant is your open source engine for controlled, compatible and targeted AI conversations-star parlant on GitHub! (Promoted)