Reasoning language models or RLMs are increasingly used to simulate step-by-step problem solving by generating long, structured reasoning chains. These models break down complex questions into simpler parts and build logical steps to reach answers. This chain-of-thought (COT) approach has been shown to be effective in improving output quality, especially in mathematical and logical tasks. Despite multilingual capabilities in many modern large models, the focus of research and training has remained largely centered in English, which has left a gap in understanding how well these reasoning skills are translated into other languages.
A major challenge is that most RLMs are fine -tuned on English data, which limits their ability to resonate effectively in other languages. This is especially problematic for low resource language that has limited training examples. The models may be standard for English thinking patterns and producing lower quality output when asked in another language. Furthermore, differences in language structure can cause reasoning errors, especially when a model trained in a language is expected to derive logic in another without sufficient linguistic adaptation.
Current techniques use zero-shot or get shots that encourage strategies to control these limitations, often using English as a turning language. Some efforts involves presenting prompts in the same language as the query to maintain linguistic consistency. However, small models have minimal benefits due to limited capacity, and even large models show inconsistent performance when they reasoning in low resource languages. Despite the multilingual pre-determination, the gap between the training and reasoning language continues to prevent accurate multilingual reasoning.
Brown University and the Mbzuai research team focused on evaluating how increasing test time calculation, especially through extended reasoning chains, can affect the multilingual reasoning skills for English-centric RLMs. They examined using S1 models based on the QWEN2.5 instruction architecture and fine-tuned on 1,000 English tribal tests. These models were tested across different languages ​​using benchmarks such as MGSM and Global-MMLU to answer four core questions: the effectiveness of cross-taking test time scaling, language mixture, performance during language author and generalization of domains.
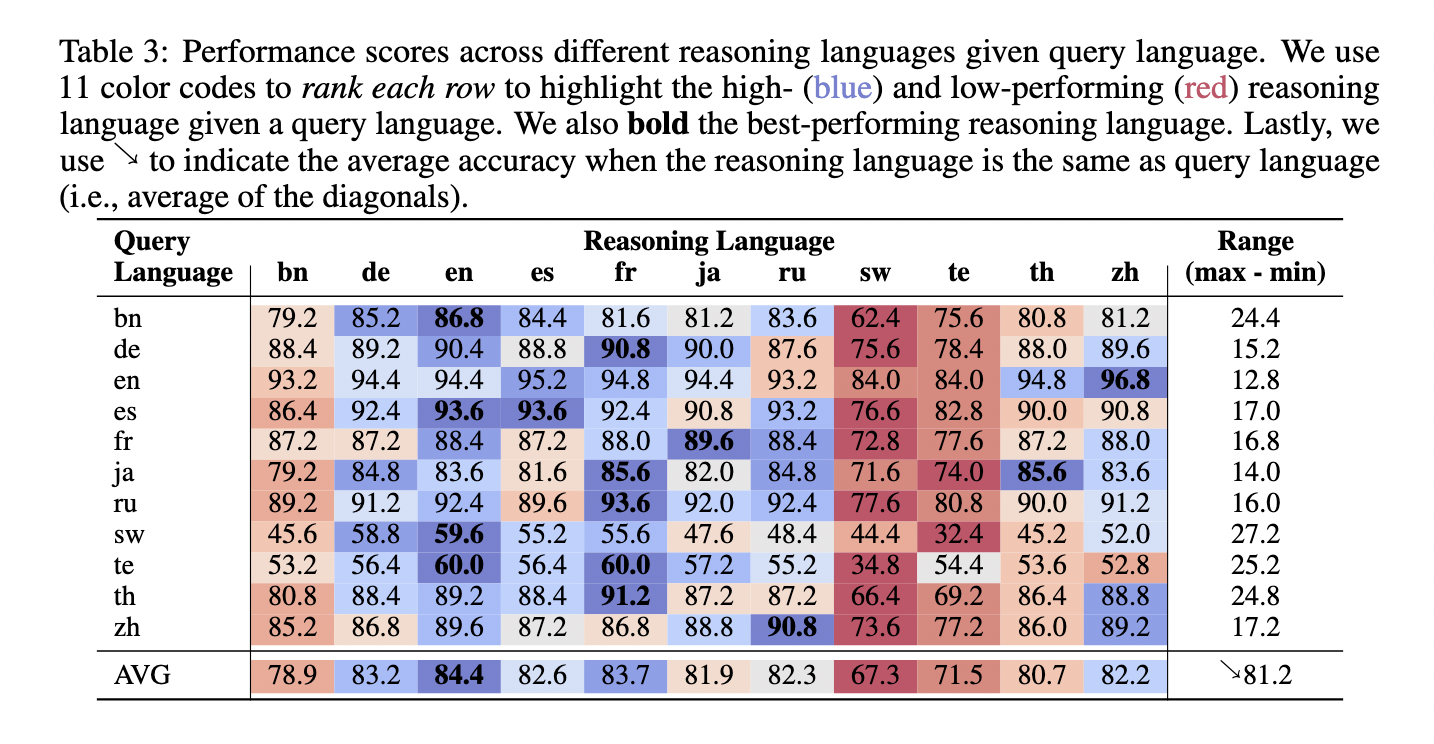
In -depth experiments showed that models with multiple parameters are significantly benefited from increased test time thinking tokens. The 14B S1 model, when it was scaled to 8,000 thinking tokens, achieved an average accuracy of 81% across non-English languages ​​in MGSM. It surpassed models such as QWEN2.5-14B instructions by +23.1% in French and +41.6% in Swahili. Although the model was only trained in English, its performance surpassed the models for larger models such as Deepseeks R1-Distill-Qwen-32B in several languages ​​with high resource. The study also found that reasoning in high resource language such as Chinese and English is more effective, requiring fewer symbols and delivers better results than in low resource languages ​​such as Swahili or Telugu.
A key observation was “quote-and-thinking” behavior, where the model quoted non-English phrases from prompt and justified in English. This consistent pattern across languages ​​such as Japanese and Russian suggested that the model use its multilingual understanding to interpret non-English input without direct translation. Language -disrupting experiments also confirmed that coercion of reasoning in high resource languages ​​produced better results, while strict reasoning in low resource language led to significant accuracy and calculation inefficiency.
Despite strong results in Stem-related tasks, performance gains did not transfer to domains such as cultural commonsense or humanities. In benchmarks as a fork, increased thinking sometimes reduced the benefit, which indicates over -thinking. The study concludes that although test time scaling improves multilingual reasoning in high resource languages, it is not effectively generalized to out-of-domain tasks or low resource languages, indicating the need for further research into balanced multilingual training and domain adaptation.
Check Paper. All credit for this research goes to the researchers in this project. You are also welcome to follow us on Twitter And don’t forget to join our 90k+ ml subbreddit.
Here is a brief overview of what we build on MarkTechpost:
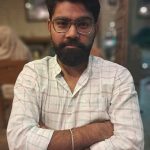
Nikhil is an internal consultant at MarkTechpost. He is pursuing an integrated double degree in materials at the Indian Institute of Technology, Kharagpur. Nikhil is an AI/ML enthusiast who always examines applications in fields such as biomaterials and biomedical science. With a strong background in material science, he explores new progress and creates opportunities to contribute.