Modern AI systems have made significant progress, yet many are still struggling with complex reasoning tasks. Questions such as inconsistent problem solving, limited chain-to-thought capabilities and occasional factual inaccuracies are left. These challenges prevent practical uses in research and software development, where nuanced understanding and precision are crucial. Driven to overcome these limitations have led to a re -examination of how AI models are built and trained, focusing on improving transparency and reliability
Xai’s recent release of Grok 3 Beta marks a thought -provoking step forward in AI development. In their message, the company outlines how this new model is based on its predecessors with a refined approach to reasoning and problem solving. GROK 3 is trained in the company’s Colossus Supercluster using significantly more calculation than previous iterations. This improved training has provided improvements in areas such as mathematics, coding and instructional, while the model can consider multiple resolution paths before arriving at a final answer.
Instead of relying on oversold promises, the release emphasizes that it is hitting 3 – and its streamlined variant, the 3 mini – is still evolving. Early access is designed to encourage user feedback, which will help guide further improvements. The model’s ability to reveal its reasoning process through a “Think” button invites users to engage directly with its problem -solving steps that promote a level of transparency that is often absent in traditional AI outputs.
Technical details and practical benefits
In its core, Grok 3 utilizes a reinforcing learning frame to improve its chain-to-thoughts. This approach allows the model to simulate a form of internal reasoning that itering over possible solutions and corrects errors along the way. Users can observe this process, which is particularly valuable in tasks where a clear reasoning is as important as the final answer. The integration of this reasoning state separates Grok 3 except for many previous models that simply generate answers without a explainable thought process.
Technically, GROK 3’s architecture benefits from an expanded context window that is now able to handle up to a million tokens. This makes it better suited for the processing of long documents and managing intricate instructions. Benchmark tests indicate remarkable improvements in various areas, including competitive math challenges, advanced reasoning tasks and code generation. For example, the model achieved a 93.3% accuracy rate of a recent math competition when it used its highest level of test time. These technical improvements are translated into practical benefits: clearer, more reliable answers that can support both academic and professional applications without unnecessary decoration.
Data insight and comparative analysis
The model’s performance in various benchmarks, such as those who evaluate reasoning and code generation, demonstrates that it can effectively handle complex tasks. Although some skepticism remains within society, the empirical results suggest that Grok 3 is a robust addition to the AI ​​landscape.
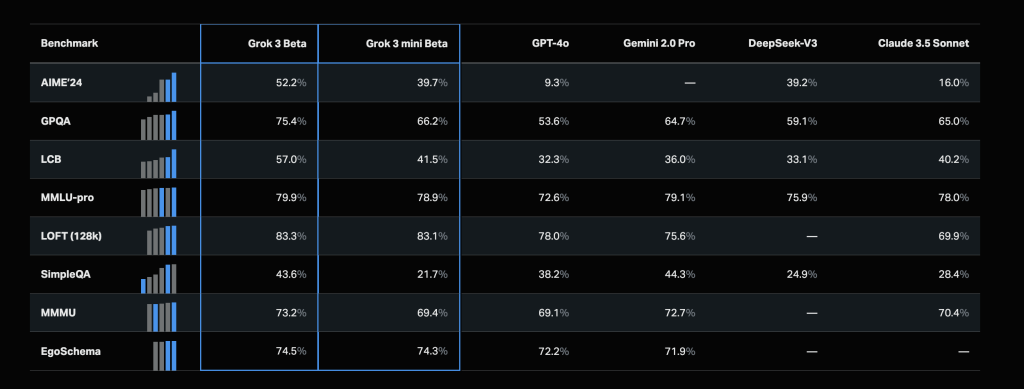
Comparative analysis with other leading models emphasizes that although many systems continue to be popular choices, Grok 3’s combination of improved reasoning and a larger context window provides a clear advantage of tackling more involved queries. In addition, the introduction of the Grok 3 mini-variant extends the range of applications by offering a more cost-effective option for tasks that do not require such extensive world knowledge. This data emphasizes the importance of continued innovation in AI, driven by strict tests and the performance of reality rather than speculative promises.
Conclusion
GROK 3 represents a thought -provoking development in search of more reliable and transparent AI Reasoning. By focusing on improved problem solving through reinforcement learning and offering users a window into its internal thought processes, the model addresses several many years of challenges. Its performance across a variety of benchmarks – ranging from competition math to advanced code generation – demonstrates that a balanced, methodological approach to AI development can provide meaningful improvements.
For researchers and developers, GROK 3 offers not only improved technical capabilities, but also a practical tool for exploring complex ideas with greater clarity. The model’s design reflects a measured progression in AI that appreciates step -by -step improvements and user engagement over hyperbolic claims. As XAI continues to refine Grok 3 based on the real world feedback, the technology is to play a significant role in both academic research and practical applications in software development.
Check out The technical details. All credit for this research goes to the researchers in this project. You are also welcome to follow us on Twitter And don’t forget to join our 75k+ ml subbreddit.
🚨 Recommended Reading AI Research Release Nexus: An Advanced System Integrating Agent AI system and Data Processing Standards To Tackle Legal Concerns In Ai Data Set
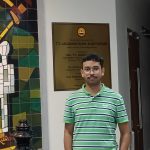
Sana Hassan, a consultant intern at MarkTechpost and dual-degree students at IIT Madras, is passionate about using technology and AI to tackle challenges in the real world. With a great interest in solving practical problems, he brings a new perspective to the intersection of AI and real solutions.
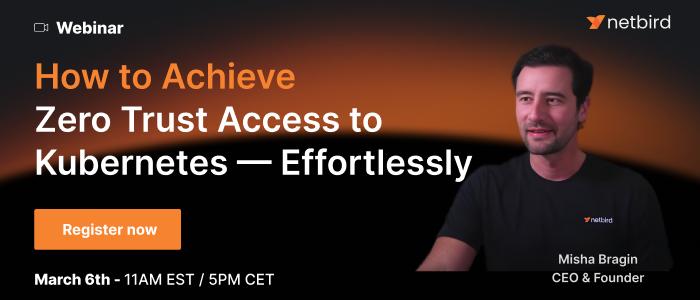