With increasing demand for AI systems that can handle tasks involving multi-step logic, mathematical evidence and software development, researchers have turned their attention to improve models’ reasoning potential. This capacity, which was once thought to be exclusive of human intelligence, is now being actively pursued in smaller models to make them more efficient and widespread. As reasoning-based tasks continue to expand in relevance, comprehensive academic problem solving, automated phrase that evidence, algorithm design and complex software discovery, language models are expected to become more than just general converts. They are encouraged to become domain -specific problem solvers who can help professionals and researchers.
A challenge in building reasoning focused models is to achieve strong, while performance in math and programming, while maintaining a relatively small model size. Most competitive results in these domains are achieved by models with approx. 32 billion parameters or more. These large models are often used because less struggles with generalization and reward optimization in reinforcing learning tasks, especially when it comes to code -based problem solving. Sparse Reward Feedback, Limited High Quality Data and Weak Basic Model Architecture make it difficult to develop compact, yet powerful models. In addition, the data used to train these models are not always curated with reasoning in mind, which often results in training inefficiency and limited gains in problem -solving skills.
To counter reasoning challenges, several models, including Openai’s O-Series, Deepseek R1 and Claude 3.7, have been introduced and utilizing massive parameter counts and complex reinforcement learning strategies. These models use techniques such as step-by-step planning and backtracking to improve reasoning, especially in algorithmic thinking and math-related tasks. However, they are highly dependent on phases after training and underplay the importance of high quality data before training. Many are also dependent on fixed template -based reward systems that tend to reward hacking. Code generation Benchmarks often reveal that these models perform inconsistent in challenging tasks due to low prior foundation foundations and inefficient reward signal modeling during fine tuning.
A research team from Xiaomi introduced MIMO-7B Family of language models with a focused approach to overcome these barriers. Innovation lies in the treatment of both pre -formation and continuing education as equally critical phases for developing reasoning capabilities. The base model, the MIMO-7B base, was trained from the bottom using a dataset that includes 25 trillion tokens. This data set was constructed with a three-step mixing strategy that gradually increased the proportion of mathematical and programming content. A further multiple-token Prediction (MTP) goal was introduced under premium to improve both performance and inference speed. For post-training, the team developed a curated data set with 130,000 verifiable math and programming problems, each labeled with difficulties. Reinforcement learning was then used using a difficulty -driven reward framework, enabling more nuanced and effective feedback during exercise. This resulted in two larger variants: MIMO-7B-RL and MIMO-7B-RL-ZERO.
The Pre-Training Metode started by extracting reasoning-heavy content from web pages, academic papers and books using a custom HTML extraction tool designed to preserve math equations and code pieces. Unlike generic pipelines, this extractor preserved structural elements that are critical to problem -solving domains. The team then improved the PDF parsing tools to interpret scientific and programming content exactly. To prevent date duplication, global deduplication was used using URL-based and minhash techniques. The training corpus was filtered using small language models fine-tuned to tag content quality and replace outdated heuristic-based filters that often removed valuable reasoning examples. High quality synthetic reasoning data was also generated from advanced models and added in the last phase of training. This three-stage approach resulted in a final training mix extensive 70% math and code data in phase two and an additional 10% of synthetic content in phase three. The maximum context length was expanded from 8,192 to 32,768 tokens, ensuring that the model could handle long form problems.
In the reinforcement learning stage, the research team constructed a trouble -free roll -out engine to speed up training and validation. This infrastructure incorporated asynchronous reward calculation and early termination mechanisms to reduce GPU gum, resulting in 2.29 times faster exercise and 1.96 times faster validation. The model’s policy was optimized using fine -grained rewards derived from the difficulty of testing cases that dealt with the sparse reward question in programming benchmarks. Re-Sampling techniques were introduced to maintain exercise stability and increase the Rollout Sampling Efficiency. Overall, these strategies made it in the MIMO-7B variants to learn effectively, even from cold starting states where there is no pre-established initialization available.
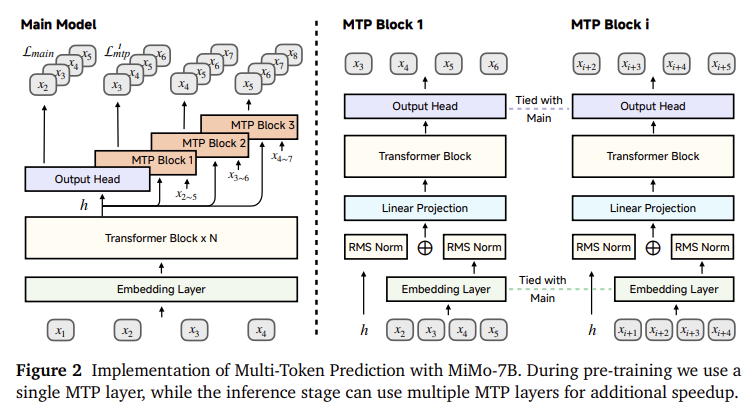
Performance evaluation revealed that MIMO-7B base achieved a score of 75.2 on the Big-Bench HARD (BBH) task, which exceeded other open source 7B models. It also worked well on Supergpqa, which includes graduate questions. The post-trained MIMO-7B-RL scored 55.4 on AIME 2025-Benchmark and exceeded Openais O1-mini with 4.7 points. On code generation tasks, it surpassed much larger models such as Deepseek-R1-Zero-32B and QWEN2.5-32B-RL-ZERO on both LIVECODEBENCH V5 and V6. These results show that a properly optimized 7B model can compete or even surpass models by more than four times the number of parameters.
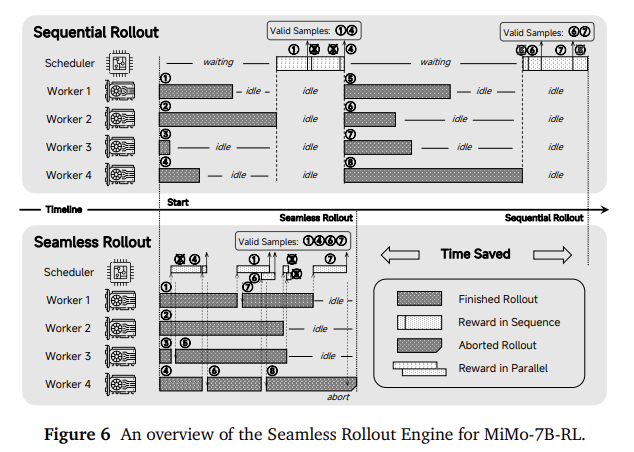
The MIMO-7B project serves as a concrete demonstration of how infrastructure for premium, data quality and reinforcement learning contributes to the final reasoning of a language model. By reconsidering the pipeline from data extraction to reward calculation, the Xiaomi research team achieved compact, yet powerful models suitable for applications in the real world in mathematics, coding and logic. Their approach highlights the unused potential for small models and challenges the assumption that size alone determines intelligence or versatility.
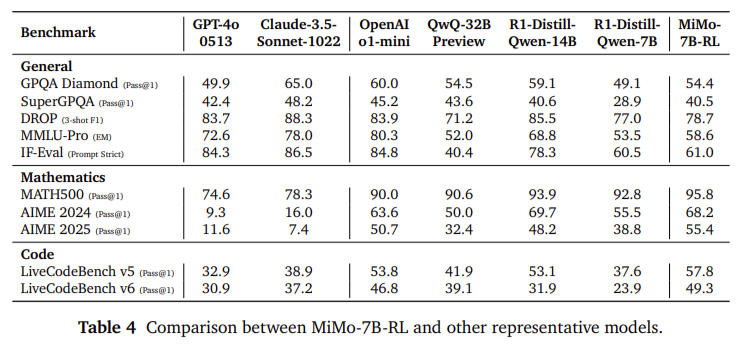
Key takeaways from the research at MIMO-7B:
- MIMO-7B was trained on a massive dataset with 25 trillion tokens that targeted reasoning tasks using structured data fiors.
- 130,000 math and code problems were used in RL training, each commented on with difficulty results to enable effective reward formation.
- Three-stage pre-training raised math and coding content to 70%, followed by 10% synthetic problem solving data.
- A hassle -free roll -out engine increased RL training speed by 2.29 times and validation by 1.96 times.
- MIMO-7B-RL achieved 55.4 on AIME 2025 and surpassed Openai O1-MINI by 4.7 points.
- MIMO-7B models are publicly available and include all control points: Base, SFT and RL variants.
- The model’s success shows that small, well-designed models can compete or exceed the performance of 32B models in reasoning tasks.
Check Paper and github side. Nor do not forget to follow us on Twitter and join in our Telegram Channel and LinkedIn GrOUP. Don’t forget to take part in our 90k+ ml subbreddit.
🔥 [Register Now] Minicon Virtual Conference On Agentic AI: Free Registration + Certificate for Participation + 4 Hours Short Event (21 May, 9- 13.00 pst) + Hands on Workshop
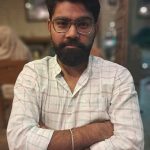
Nikhil is an internal consultant at MarkTechpost. He is pursuing an integrated double degree in materials at the Indian Institute of Technology, Kharagpur. Nikhil is an AI/ML enthusiast who always examines applications in fields such as biomaterials and biomedical science. With a strong background in material science, he explores new progress and creates opportunities to contribute.
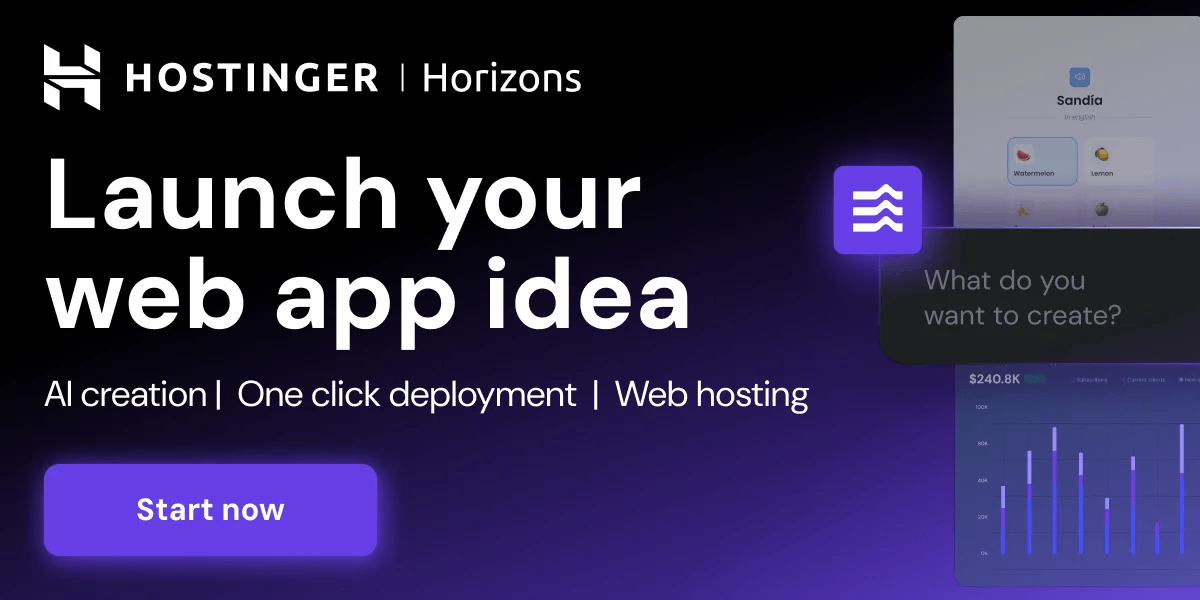